
Sorenson Squeeze Compression Suite v4.2.301 6 serial key or number
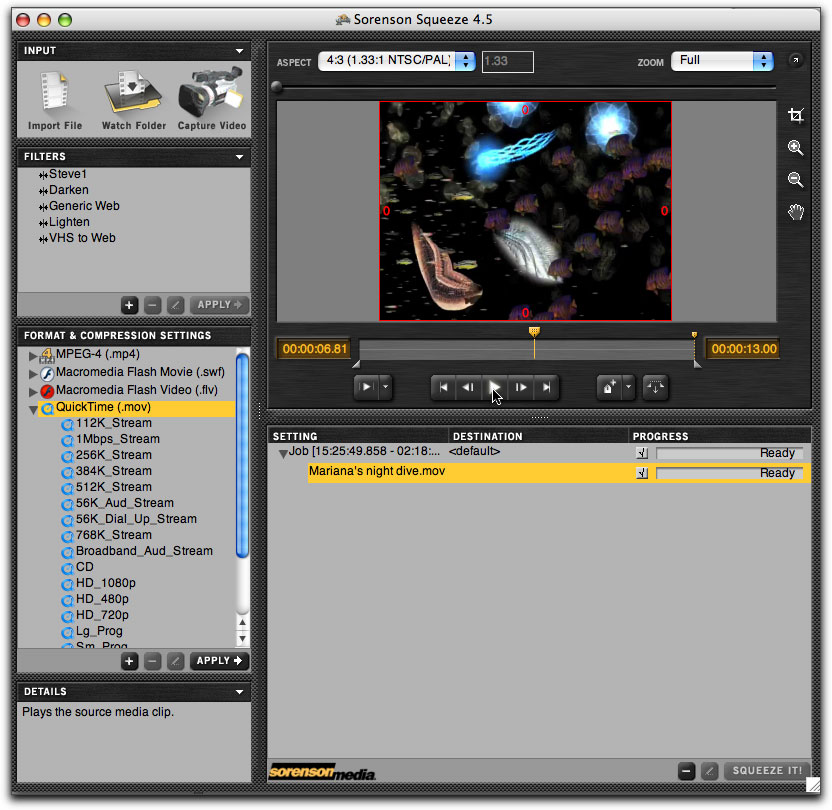
Sorenson Squeeze Compression Suite v4.2.301 6 serial key or number
TS-G-1.1 (ST-2) - RAMPAC
IAEA
SAFETY
STANDARDS
SERIES
Advisory Material for the
IAEA Regulations for the
Safe Transport of
Radioactive Material
SAFETY GUIDE
No. TS-G-1.1 (ST-2)
INTERNATIONAL
ATOMIC ENERGY AGENCY
VIENNA
IAEA SAFETY RELATED PUBLICATIONS
IAEA SAFETY STANDARDS
Under the terms of Article III of its Statute, the IAEA is authorized to establish standards
of safety for protection against ionizing radiation and to provide for the application of these
standards to peaceful nuclear activities.
The regulatory related publications by means of which the IAEA establishes safety
standards and measures are issued in the IAEA Safety Standards Series. This series covers
nuclear safety, radiation safety, transport safety and waste safety, and also general safety (that
is, of relevance in two or more of the four areas), and the categories within it are Safety
Fundamentals, Safety Requirements and Safety Guides.
Safety Fundamentals (blue lettering) present basic objectives, concepts and principles of
safety and protection in the development and application of nuclear energy for peaceful
purposes.
Safety Requirements (red lettering) establish the requirements that must be met to ensure
safety. These requirements, which are expressed as ‘shall’ statements, are governed by
the objectives and principles presented in the Safety Fundamentals.
Safety Guides (green lettering) recommend actions, conditions or procedures for meeting
safety requirements. Recommendations in Safety Guides are expressed as ‘should’ statements,
with the implication that it is necessary to take the measures recommended or
equivalent alternative measures to comply with the requirements.
The IAEA’s safety standards are not legally binding on Member States but may be
adopted by them, at their own discretion, for use in national regulations in respect of their own
activities. The standards are binding on the IAEA in relation to its own operations and on States
in relation to operations assisted by the IAEA.
Information on the IAEA’s safety standards programme (including editions in languages
other than English) is available at the IAEA Internet site
www.iaea.org/ns/coordinet
or on request to the Safety Co-ordination Section, IAEA, P.O. Box 100, A-1400 Vienna, Austria.
OTHER SAFETY RELATED PUBLICATIONS
Under the terms of Articles III and VIII.C of its Statute, the IAEA makes available and
fosters the exchange of information relating to peaceful nuclear activities and serves as an intermediary
among its Member States for this purpose.
Reports on safety and protection in nuclear activities are issued in other series, in
particular the IAEA Safety Reports Series, as informational publications. Safety Reports may
describe good practices and give practical examples and detailed methods that can be used to
meet safety requirements. They do not establish requirements or make recommendations.
Other IAEA series that include safety related sales publications are the Technical
Reports Series, the Radiological Assessment Reports Series and the INSAG Series. The
IAEA also issues reports on radiological accidents and other special sales publications.
Unpriced safety related publications are issued in the TECDOC Series, the Provisional Safety
Standards Series, the Training Course Series, the IAEA Services Series and the Computer
Manual Series, and as Practical Radiation Safety Manuals and Practical Radiation
Technical Manuals.
ADVISORY MATERIAL FOR THE
IAEA REGULATIONS FOR THE
SAFE TRANSPORT OF
RADIOACTIVE MATERIAL
The following States are Members of the International Atomic Energy Agency:
AFGHANISTAN
ALBANIA
ALGERIA
ANGOLA
ARGENTINA
ARMENIA
AUSTRALIA
AUSTRIA
AZERBAIJAN
BANGLADESH
BELARUS
BELGIUM
BENIN
BOLIVIA
BOSNIA AND HERZEGOVINA
BOTSWANA
BRAZIL
BULGARIA
BURKINA FASO
CAMBODIA
CAMEROON
CANADA
CENTRAL AFRICAN
REPUBLIC
CHILE
CHINA
COLOMBIA
COSTA RICA
CÔTE D’IVOIRE
CROATIA
CUBA
CYPRUS
CZECH REPUBLIC
DEMOCRATIC REPUBLIC
OF THE CONGO
DENMARK
DOMINICAN REPUBLIC
ECUADOR
EGYPT
EL SALVADOR
ESTONIA
ETHIOPIA
FINLAND
FRANCE
GABON
GEORGIA
GERMANY
GHANA
GREECE
GUATEMALA
HAITI
HOLY SEE
HUNGARY
ICELAND
INDIA
INDONESIA
IRAN, ISLAMIC REPUBLIC OF
IRAQ
IRELAND
ISRAEL
ITALY
JAMAICA
JAPAN
JORDAN
KAZAKHSTAN
KENYA
KOREA, REPUBLIC OF
KUWAIT
LATVIA
LEBANON
LIBERIA
LIBYAN ARAB JAMAHIRIYA
LIECHTENSTEIN
LITHUANIA
LUXEMBOURG
MADAGASCAR
MALAYSIA
MALI
MALTA
MARSHALL ISLANDS
MAURITIUS
MEXICO
MONACO
MONGOLIA
MOROCCO
MYANMAR
NAMIBIA
NETHERLANDS
NEW ZEALAND
NICARAGUA
NIGER
NIGERIA
NORWAY
PAKISTAN
PANAMA
PARAGUAY
PERU
PHILIPPINES
POLAND
PORTUGAL
QATAR
REPUBLIC OF MOLDOVA
ROMANIA
RUSSIAN FEDERATION
SAUDI ARABIA
SENEGAL
SIERRA LEONE
SINGAPORE
SLOVAKIA
SLOVENIA
SOUTH AFRICA
SPAIN
SRI LANKA
SUDAN
SWEDEN
SWITZERLAND
SYRIAN ARAB REPUBLIC
TAJIKISTAN
THAILAND
THE FORMER YUGOSLAV
REPUBLIC OF MACEDONIA
TUNISIA
TURKEY
UGANDA
UKRAINE
UNITED ARAB EMIRATES
UNITED KINGDOM OF
GREAT BRITAIN AND
NORTHERN IRELAND
UNITED REPUBLIC
OF TANZANIA
UNITED STATES OF AMERICA
URUGUAY
UZBEKISTAN
VENEZUELA
VIET NAM
YEMEN
YUGOSLAVIA,
FEDERAL REPUBLIC OF
ZAMBIA
ZIMBABWE
The Agency’s Statute was approved on 23 October 1956 by the Conference on the Statute of the
IAEA held at United Nations Headquarters, New York; it entered into force on 29 July 1957. The
Headquarters of the Agency are situated in Vienna. Its principal objective is “to accelerate and enlarge the
contribution of atomic energy to peace, health and prosperity throughout the world’’.
© IAEA, 2002
Permission to reproduce or translate the information contained in this publication may be
obtained by writing to the International Atomic Energy Agency, Wagramer Strasse 5, P.O. Box 100,
A-1400 Vienna, Austria.
Printed by the IAEA in Austria
June 2002
STI/PUB/1109
SAFETY STANDARDS SERIES No. TS-G-1.1 (ST-2)
ADVISORY MATERIAL FOR THE
IAEA REGULATIONS FOR THE
SAFE TRANSPORT OF
RADIOACTIVE MATERIAL
SAFETY GUIDE
INTERNATIONAL ATOMIC ENERGY AGENCY
VIENNA, 2002
VIC Library Cataloguing in Publication Data
Advisory material for the IAEA regulations for the safe transport of radioactive
material : safety guide. — Vienna : International Atomic Energy Agency,
2002.
p. ; 24 cm. — (Safety standards series, ISSN 1020–525X;
no. TS-G-1.1 (ST-2))
STI/PUB/1109
ISBN 92–0–111802–3
Includes bibliographical references.
1. Radioactive substances — Transportation — Safety regulations.
I. International Atomic Energy Agency. II. Series.
VICL 02–00271
FOREWORD
by Mohamed ElBaradei
Director General
One of the statutory functions of the IAEA is to establish or adopt standards of
safety for the protection of health, life and property in the development and application
of nuclear energy for peaceful purposes, and to provide for the application of these
standards to its own operations as well as to assisted operations and, at the request of
the parties, to operations under any bilateral or multilateral arrangement, or, at the
request of a State, to any of that State’s activities in the field of nuclear energy.
The following bodies oversee the development of safety standards: the
Commission for Safety Standards (CSS); the Nuclear Safety Standards Committee
(NUSSC); the Radiation Safety Standards Committee (RASSC); the Transport Safety
Standards Committee (TRANSSC); and the Waste Safety Standards Committee
(WASSC). Member States are widely represented on these committees.
In order to ensure the broadest international consensus, safety standards are
also submitted to all Member States for comment before approval by the IAEA Board
of Governors (for Safety Fundamentals and Safety Requirements) or, on behalf of the
Director General, by the Publications Committee (for Safety Guides).
The IAEA’s safety standards are not legally binding on Member States but may
be adopted by them, at their own discretion, for use in national regulations in respect
of their own activities. The standards are binding on the IAEA in relation to its own
operations and on States in relation to operations assisted by the IAEA. Any State
wishing to enter into an agreement with the IAEA for its assistance in connection
with the siting, design, construction, commissioning, operation or decommissioning
of a nuclear facility or any other activities will be required to follow those parts of the
safety standards that pertain to the activities to be covered by the agreement.
However, it should be recalled that the final decisions and legal responsibilities in any
licensing procedures rest with the States.
Although the safety standards establish an essential basis for safety, the
incorporation of more detailed requirements, in accordance with national practice,
may also be necessary. Moreover, there will generally be special aspects that need to
be assessed on a case by case basis.
The physical protection of fissile and radioactive materials and of nuclear
power plants as a whole is mentioned where appropriate but is not treated in detail;
obligations of States in this respect should be addressed on the basis of the relevant
instruments and publications developed under the auspices of the IAEA. Nonradiological
aspects of industrial safety and environmental protection are also not
explicitly considered; it is recognized that States should fulfil their international
undertakings and obligations in relation to these.
The requirements and recommendations set forth in the IAEA safety standards
might not be fully satisfied by some facilities built to earlier standards. Decisions on
the way in which the safety standards are applied to such facilities will be taken by
individual States.
The attention of States is drawn to the fact that the safety standards of the
IAEA, while not legally binding, are developed with the aim of ensuring that the
peaceful uses of nuclear energy and of radioactive materials are undertaken in a
manner that enables States to meet their obligations under generally accepted
principles of international law and rules such as those relating to environmental
protection. According to one such general principle, the territory of a State must not
be used in such a way as to cause damage in another State. States thus have an
obligation of diligence and standard of care.
Civil nuclear activities conducted within the jurisdiction of States are, as any
other activities, subject to obligations to which States may subscribe under
international conventions, in addition to generally accepted principles of international
law. States are expected to adopt within their national legal systems such legislation
(including regulations) and other standards and measures as may be necessary to fulfil
all of their international obligations effectively.
EDITORIAL NOTE
An appendix, when included, is considered to form an integral part of the standard and
to have the same status as the main text. Annexes, footnotes and bibliographies, if included, are
used to provide additional information or practical examples that might be helpful to the user.
The safety standards use the form ‘shall’ in making statements about requirements,
responsibilities and obligations. Use of the form ‘should’ denotes recommendations of a
desired option.
The English version of the text is the authoritative version.
PREFACE
This Advisory Material is not a stand-alone text. It only has significance when
used concurrently as a companion to the IAEA Safety Standards Series No. ST-1 1 ,
Regulations for the Safe Transport of Radioactive Material (1996 edition), denoted
henceforth as “the Regulations”. To facilitate cross-reference between it and the
Regulations, each paragraph of the Advisory Material is numbered in correspondence
with the paragraph of the Regulations to which it most directly relates. To distinguish
paragraphs of the Advisory Material from those of the Regulations for reference
purposes, Advisory Material paragraphs always have a numeral after the decimal
point, even when there is only one subparagraph of text. Thus, for example, advice
relating to para. 401 of the Regulations should be initially sought under para. 401.1
of the Advisory Material. Integral paragraph numbers which are cited in the text,
either alone or accompanied by lower case letters in brackets, should be taken as
identifying paragraphs of the Regulations.
Also, the publications listed under “References” are the versions which were
used in the development of the 1996 edition of the Regulations and this Advisory
Material. Some of the publications may have been superseded by later revisions.
These may be consulted for the most recent information recognizing that the earlier
editions are the basis for the discussions which follow.
Since the first edition in 1961, the Regulations for the Safe Transport of
Radioactive Material of the International Atomic Energy Agency (IAEA Regulations)
have served as the basis of safety for the transport of radioactive material worldwide.
The provisions of the IAEA Regulations have been adopted in national regulations by
most of the Member States of the Agency. The international regulatory bodies having
responsibility for the various modes of transport have also implemented the IAEA
Regulations. The safety record since the inception, and throughout several
comprehensive revisions, of the Regulations has demonstrated the efficacy both of the
regulatory provisions and of the arrangements for ensuring compliance with them.
In the discussions leading to the first edition of the IAEA Regulations, it was
realized that there was need for a publication to supplement the Regulations which
could give information on individual provisions as to their purpose, their scientific
background and how to apply them in practice. The scientific basis of the
classification of radioisotopes for transport purposes, then in use, and the factors that
1
The Regulations for the Safe Transport of Radioactive Material were issued in 1996
as Safety Standards Series No. ST-1. In 2000 the Regulations were issued in English, with
minor editorial corrections, as Safety Standards Series No. TS-R-1 (ST-1, Revised).
have to be taken into account by competent authorities in approving package designs,
were examples adduced in support of this concept at the time. In response, the Agency
published Safety Series No. 7, entitled, in its first edition in 1961, “Notes on Certain
Aspects of the Regulations”.
As experience in applying the Regulations grew, it became increasingly evident
that, while the provisions of the Regulations might be essentially clear and
unambiguous, nevertheless they would often also be highly technical in nature and
unavoidably complex. Moreover they intentionally state no more than ‘what’ must be
achieved in relation to package characteristics and operational conditions in order to
assure safety. They do not seek to prescribe ‘how’ the user should comply; indeed the
freedom to innovate and to develop new ways to ensure compliance is recognized as
intrinsically desirable in such a technically advanced field. An additional source of
information on the Regulations, providing advice on ‘how’ to comply with them
which could be augmented from time to time in the light of latest experience, was
therefore provided by the Agency, initially in relation to the 1973 edition of the
Regulations. This was entitled “Advisory Material for the IAEA Regulations for the
Safe Transport of Radioactive Material”. It was designated Safety Series No. 37.
Up to the time of publication of the previous edition of the IAEA Regulations,
in 1985, Safety Series No. 37 had reached its third edition. Meanwhile, Safety Series
No. 7, which embodied information on the scientific basis and rationale of the
Regulations, had been retitled “Explanatory Material for the IAEA Regulations for
the Safe Transport of Radioactive Material” and, embodying mainly information on
the scientific basis and rationale of the Regulations, was in its second edition.
During the current regulatory revision, which culminated in 1996, the Agency’s
senior advisory body for transport, the Transport Safety Standards Advisory
Committee (TRANSSAC), in consultation with the Agency’s Publishing Section,
agreed that it would be a useful simplification to combine the two Safety Guides
previously known as Safety Series No. 7 and Safety Series No. 37 in a single
publication, to be known as “Advisory Material for the IAEA Regulations for the Safe
Transport of Radioactive Material”. This would have the advantage of consolidating
supporting information on the Regulations in one place, eliminating duplication. The
advisory nature of the present publication has been made paramount. The inclusion
of some explanatory material supports this function since a proper understanding of
the background to the regulatory provisions helps users to interpret them correctly
and to comply with them fully.
Thus the primary purpose of this publication (henceforth referred to as the
Advisory Material) is to provide guidance to users on proven and acceptable ways of
complying with and demonstrating compliance with the Regulations. It must be
emphasized that the text is not to be construed as being uniquely prescriptive. It offers
recommendations on ways of complying but it does not lay down ‘the only way’ to
comply with any specific provision.
Member States and international organizations are invited to take note of this
publication and to bring it to the attention of persons and organizations who make use
of, or are subject to, the IAEA Regulations. Moreover, readers are encouraged to
send, through their competent authority, any comments they may wish to make,
including proposals for modifications, additions or deletions, to the International
Atomic Energy Agency.
CONTENTS
SECTION I. INTRODUCTION . . . . . . . . . . . . . . . . . . . . . . . . . . . . . . . . 1
Background . . . . . . . . . . . . . . . . . . . . . . . . . . . . . . . . . . . . . . . . . . . . . 1
Objective . . . . . . . . . . . . . . . . . . . . . . . . . . . . . . . . . . . . . . . . . . . . . . . 1
Scope . . . . . . . . . . . . . . . . . . . . . . . . . . . . . . . . . . . . . . . . . . . . . . . . . . 1
Reference to Section I . . . . . . . . . . . . . . . . . . . . . . . . . . . . . . . . . . . . . 3
SECTION II. DEFINITIONS . . . . . . . . . . . . . . . . . . . . . . . . . . . . . . . . . . . 5
References to Section II . . . . . . . . . . . . . . . . . . . . . . . . . . . . . . . . . . . . 28
SECTION III. GENERAL PROVISIONS . . . . . . . . . . . . . . . . . . . . . . . . . . 29
Radiation protection . . . . . . . . . . . . . . . . . . . . . . . . . . . . . . . . . . . . . . . 29
Emergency response . . . . . . . . . . . . . . . . . . . . . . . . . . . . . . . . . . . . . . . 34
Quality assurance . . . . . . . . . . . . . . . . . . . . . . . . . . . . . . . . . . . . . . . . . 35
Compliance assurance . . . . . . . . . . . . . . . . . . . . . . . . . . . . . . . . . . . . . 36
Special arrangement . . . . . . . . . . . . . . . . . . . . . . . . . . . . . . . . . . . . . . . 38
References to Section III . . . . . . . . . . . . . . . . . . . . . . . . . . . . . . . . . . . 39
SECTION IV.
ACTIVITY LIMITS AND MATERIAL
RESTRICTIONS . . . . . . . . . . . . . . . . . . . . . . . . . . . . . . . . . 41
Basic radionuclide values . . . . . . . . . . . . . . . . . . . . . . . . . . . . . . . . . . 41
Determination of basic radionuclide values . . . . . . . . . . . . . . . . . . . . . 43
Contents limits for packages . . . . . . . . . . . . . . . . . . . . . . . . . . . . . . . . . 44
References to Section IV . . . . . . . . . . . . . . . . . . . . . . . . . . . . . . . . . . . 48
SECTION V.
REQUIREMENTS AND CONTROLS
FOR TRANSPORT . . . . . . . . . . . . . . . . . . . . . . . . . . . . . . . 51
Requirements before the first shipment . . . . . . . . . . . . . . . . . . . . . . . . . 51
Requirements before each shipment . . . . . . . . . . . . . . . . . . . . . . . . . . . 53
Transport of other goods . . . . . . . . . . . . . . . . . . . . . . . . . . . . . . . . . . . . 55
Other dangerous properties of contents . . . . . . . . . . . . . . . . . . . . . . . . . 56
Requirements and controls for contamination and
for leaking packages . . . . . . . . . . . . . . . . . . . . . . . . . . . . . . . . . . . . 57
Requirements and controls for transport of excepted packages . . . . . . . 63
Requirements and controls for transport of LSA material and SCO
in industrial packages or unpackaged . . . . . . . . . . . . . . . . . . . . . . . 67
Determination of transport index . . . . . . . . . . . . . . . . . . . . . . . . . . . . . 68
Determination of criticality safety index . . . . . . . . . . . . . . . . . . . . . . . . 70
Limits on transport index, criticality safety index and
radiation levels for packages and overpacks . . . . . . . . . . . . . . . . . . . 71
Categories . . . . . . . . . . . . . . . . . . . . . . . . . . . . . . . . . . . . . . . . . . . . . . 71
Marking, labelling and placarding . . . . . . . . . . . . . . . . . . . . . . . . . . . . . 73
Consignor’s responsibilities . . . . . . . . . . . . . . . . . . . . . . . . . . . . . . . . . 79
Transport and storage in transit . . . . . . . . . . . . . . . . . . . . . . . . . . . . . . . 82
Customs operations . . . . . . . . . . . . . . . . . . . . . . . . . . . . . . . . . . . . . . . 93
Undeliverable consignments . . . . . . . . . . . . . . . . . . . . . . . . . . . . . . . . . 94
References to Section V . . . . . . . . . . . . . . . . . . . . . . . . . . . . . . . . . . . . 94
SECTION VI.
REQUIREMENTS FOR RADIOACTIVE
MATERIALS AND FOR PACKAGINGS
AND PACKAGES . . . . . . . . . . . . . . . . . . . . . . . . . . . . . . . . 97
Requirements for radioactive materials . . . . . . . . . . . . . . . . . . . . . . . . . 97
General requirements for all packagings and packages . . . . . . . . . . . . . 101
Additional requirements for packages transported by air . . . . . . . . . . . . 104
Requirements for excepted packages . . . . . . . . . . . . . . . . . . . . . . . . . . 105
Requirements for industrial packages . . . . . . . . . . . . . . . . . . . . . . . . . . 105
Requirements for packages containing uranium hexafluoride . . . . . . . . 110
Requirements for Type A packages . . . . . . . . . . . . . . . . . . . . . . . . . . . . 113
Requirements for Type B(U) packages . . . . . . . . . . . . . . . . . . . . . . . . . 119
Requirements for Type B(M) packages . . . . . . . . . . . . . . . . . . . . . . . . . 134
Requirements for Type C packages . . . . . . . . . . . . . . . . . . . . . . . . . . . . 136
Requirements for packages containing fissile material . . . . . . . . . . . . . 137
References to Section VI . . . . . . . . . . . . . . . . . . . . . . . . . . . . . . . . . . . 148
SECTION VII. TEST PROCEDURES . . . . . . . . . . . . . . . . . . . . . . . . . . . . . 153
Demonstration of compliance . . . . . . . . . . . . . . . . . . . . . . . . . . . . . . . . 153
Tests for special form radioactive material . . . . . . . . . . . . . . . . . . . . . . 160
Tests for low dispersible radioactive material . . . . . . . . . . . . . . . . . . . . 162
Tests for packages . . . . . . . . . . . . . . . . . . . . . . . . . . . . . . . . . . . . . . . . 163
References to Section VII . . . . . . . . . . . . . . . . . . . . . . . . . . . . . . . . . . . 194
SECTION VIII. APPROVAL AND ADMINISTRATIVE
REQUIREMENTS . . . . . . . . . . . . . . . . . . . . . . . . . . . . . . . . 199
General aspects . . . . . . . . . . . . . . . . . . . . . . . . . . . . . . . . . . . . . . . . . . . 199
Approval of special form radioactive material and
low dispersible radioactive material . . . . . . . . . . . . . . . . . . . . . . . . . 200
Approval of package designs . . . . . . . . . . . . . . . . . . . . . . . . . . . . . . . . 200
Transitional arrangements . . . . . . . . . . . . . . . . . . . . . . . . . . . . . . . . . . . 202
Notification and registration of serial numbers . . . . . . . . . . . . . . . . . . . 206
Approval of shipments . . . . . . . . . . . . . . . . . . . . . . . . . . . . . . . . . . . . . 207
Approval of shipments under special arrangement . . . . . . . . . . . . . . . . 208
Competent authority approval certificates . . . . . . . . . . . . . . . . . . . . . . . 209
Contents of approval certificates . . . . . . . . . . . . . . . . . . . . . . . . . . . . . . 210
Validation of certificates . . . . . . . . . . . . . . . . . . . . . . . . . . . . . . . . . . . 213
Reference to Section VIII . . . . . . . . . . . . . . . . . . . . . . . . . . . . . . . . . . . 214
APPENDIX I:
THE Q SYSTEM FOR THE CALCULATION AND
APPLICATION OF A 1
AND A 2
VALUES . . . . . . . . . . . . . . 215
Introduction . . . . . . . . . . . . . . . . . . . . . . . . . . . . . . . . . . . . . . . . . . . . . 215
Background . . . . . . . . . . . . . . . . . . . . . . . . . . . . . . . . . . . . . . . . . . . . . 216
Basis of the Q system . . . . . . . . . . . . . . . . . . . . . . . . . . . . . . . . . . . . . . 217
Dosimetric models and assumptions . . . . . . . . . . . . . . . . . . . . . . . . . . . 219
Special considerations . . . . . . . . . . . . . . . . . . . . . . . . . . . . . . . . . . . . . 229
Applications . . . . . . . . . . . . . . . . . . . . . . . . . . . . . . . . . . . . . . . . . . . . . 233
Tabulation of Q values . . . . . . . . . . . . . . . . . . . . . . . . . . . . . . . . . . . . . 237
Decay chains used in the Q system . . . . . . . . . . . . . . . . . . . . . . . . . . . . 252
Conclusions . . . . . . . . . . . . . . . . . . . . . . . . . . . . . . . . . . . . . . . . . . . . . 252
References to Appendix I . . . . . . . . . . . . . . . . . . . . . . . . . . . . . . . . . . . 255
APPENDIX II:
HALF-LIFE AND SPECIFIC ACTIVITY OF
RADIONUCLIDES, DOSE AND DOSE RATE
COEFFICIENTS OF RADIONUCLIDES AND
SPECIFIC ACTIVITY . . . . . . . . . . . . . . . . . . . . . . . . . . . . . 259
Reference to Appendix II . . . . . . . . . . . . . . . . . . . . . . . . . . . . . . . . . . . 285
APPENDIX III: EXAMPLE CALCULATIONS FOR
ESTABLISHING MINIMUM SEGREGATION
DISTANCE REQUIREMENTS . . . . . . . . . . . . . . . . . . . . . . 287
Introduction . . . . . . . . . . . . . . . . . . . . . . . . . . . . . . . . . . . . . . . . . . . . . 287
Below main deck stowage of one group of packages
in passenger aircraft . . . . . . . . . . . . . . . . . . . . . . . . . . . . . . . . . . . . 289
Below main deck stowage of multiple groups of packages
in passenger aircraft . . . . . . . . . . . . . . . . . . . . . . . . . . . . . . . . . . . . 292
Main deck stowage on combi or cargo aircraft . . . . . . . . . . . . . . . . . . . 294
Segregation distances for undeveloped film . . . . . . . . . . . . . . . . . . . . . 295
References to Appendix III . . . . . . . . . . . . . . . . . . . . . . . . . . . . . . . . . . 295
APPENDIX IV: QUALITY ASSURANCE IN THE SAFE TRANSPORT
OF RADIOACTIVE MATERIAL . . . . . . . . . . . . . . . . . . . . 297
Introduction . . . . . . . . . . . . . . . . . . . . . . . . . . . . . . . . . . . . . . . . . . . . . 297
Quality assurance programmes . . . . . . . . . . . . . . . . . . . . . . . . . . . . . . . 301
Organization . . . . . . . . . . . . . . . . . . . . . . . . . . . . . . . . . . . . . . . . . . . . . 302
Document control . . . . . . . . . . . . . . . . . . . . . . . . . . . . . . . . . . . . . . . . . 303
Design control . . . . . . . . . . . . . . . . . . . . . . . . . . . . . . . . . . . . . . . . . . . 304
Procurement control . . . . . . . . . . . . . . . . . . . . . . . . . . . . . . . . . . . . . . . 306
Material control . . . . . . . . . . . . . . . . . . . . . . . . . . . . . . . . . . . . . . . . . . 307
Process control . . . . . . . . . . . . . . . . . . . . . . . . . . . . . . . . . . . . . . . . . . . 307
Inspection and test control . . . . . . . . . . . . . . . . . . . . . . . . . . . . . . . . . . 308
Non-conformity control . . . . . . . . . . . . . . . . . . . . . . . . . . . . . . . . . . . . 310
Corrective actions . . . . . . . . . . . . . . . . . . . . . . . . . . . . . . . . . . . . . . . . . 310
Records . . . . . . . . . . . . . . . . . . . . . . . . . . . . . . . . . . . . . . . . . . . . . . . . 310
Staff and training . . . . . . . . . . . . . . . . . . . . . . . . . . . . . . . . . . . . . . . . . 311
Servicing . . . . . . . . . . . . . . . . . . . . . . . . . . . . . . . . . . . . . . . . . . . . . . . 311
Audits . . . . . . . . . . . . . . . . . . . . . . . . . . . . . . . . . . . . . . . . . . . . . . . . . 311
Definitions of terms used in Appendix IV . . . . . . . . . . . . . . . . . . . . . . . 312
References to Appendix IV . . . . . . . . . . . . . . . . . . . . . . . . . . . . . . . . . . 313
APPENDIX V:
PACKAGE STOWAGE AND RETENTION
DURING TRANSPORT . . . . . . . . . . . . . . . . . . . . . . . . . . . . 315
Introduction . . . . . . . . . . . . . . . . . . . . . . . . . . . . . . . . . . . . . . . . . . . . . 315
Types of retention system . . . . . . . . . . . . . . . . . . . . . . . . . . . . . . . . . . 315
Package acceleration factor considerations . . . . . . . . . . . . . . . . . . . . . . 317
Demonstrating compliance through testing . . . . . . . . . . . . . . . . . . . . . . 319
Examples of retention system designs and assessments . . . . . . . . . . . . . 320
Definitions of terms used in Appendix V . . . . . . . . . . . . . . . . . . . . . . . 326
References to Appendix V . . . . . . . . . . . . . . . . . . . . . . . . . . . . . . . . . . 327
APPENDIX VI: GUIDELINES FOR SAFE DESIGN OF SHIPPING
PACKAGES AGAINST BRITTLE FRACTURE . . . . . . . . . 329
Introduction . . . . . . . . . . . . . . . . . . . . . . . . . . . . . . . . . . . . . . . . . . . . . 329
General consideration of evaluation methods . . . . . . . . . . . . . . . . . . . . 330
Considerations for fracture mechanics . . . . . . . . . . . . . . . . . . . . . . . . . 334
Safety factors for Method 3 . . . . . . . . . . . . . . . . . . . . . . . . . . . . . . . . . 337
Evaluation procedure for Method 3 . . . . . . . . . . . . . . . . . . . . . . . . . . . . 339
References to Appendix VI . . . . . . . . . . . . . . . . . . . . . . . . . . . . . . . . . . 344
APPENDIX VII: CRITICALITY SAFETY ASSESSMENTS . . . . . . . . . . . . . 347
Introduction . . . . . . . . . . . . . . . . . . . . . . . . . . . . . . . . . . . . . . . . . . . . . 347
Package description . . . . . . . . . . . . . . . . . . . . . . . . . . . . . . . . . . . . . . . 347
Criticality safety analysis models . . . . . . . . . . . . . . . . . . . . . . . . . . . . . 348
Method of analysis . . . . . . . . . . . . . . . . . . . . . . . . . . . . . . . . . . . . . . . . 350
Validation of calculational method . . . . . . . . . . . . . . . . . . . . . . . . . . . . 352
Calculations and results . . . . . . . . . . . . . . . . . . . . . . . . . . . . . . . . . . . . 358
Special issues . . . . . . . . . . . . . . . . . . . . . . . . . . . . . . . . . . . . . . . . . . . . 365
Design and operational issues . . . . . . . . . . . . . . . . . . . . . . . . . . . . . . . . 368
References to Appendix VII . . . . . . . . . . . . . . . . . . . . . . . . . . . . . . . . . 370
CONTRIBUTORS TO DRAFTING AND REVIEW . . . . . . . . . . . . . . . . . . . . 375
BODIES FOR THE ENDORSEMENT OF SAFETY STANDARDS . . . . . . . . 380
INDEX . . . . . . . . . . . . . . . . . . . . . . . . . . . . . . . . . . . . . . . . . . . . . . . . . . . . . . 382
LIST OF TABLES
Table I Correction factors for package and detector sizes . . . . . . . . . 22
Table II Sample segregation between classes of dangerous goods . . . 85
Table III Comparison of the four volumetric leak test
methods recommended by Aston et al. [3] . . . . . . . . . . . 98
Table IV List of VRI codes by country . . . . . . . . . . . . . . . . . . . . . . . . 211
Table I.1 Dose coefficients for submersion . . . . . . . . . . . . . . . . . . . . . 229
Table I.2 Type A package contents limits . . . . . . . . . . . . . . . . . . . . . . 238
Table I.3 Decay chains used in the Q system . . . . . . . . . . . . . . . . . . . . 253
Table II.1 Half-life and specific activity of radionuclides . . . . . . . . . . . 259
Table II.2 Dose and dose rate coefficients of radionuclides . . . . . . . . . . 272
Table II.3
Specific activity values for uranium at various
levels of enrichment . . . . . . . . . . . . . . . . . . . . . . . . . . . . 286
Table III.1 Transmission factors . . . . . . . . . . . . . . . . . . . . . . . . . . . . . . . 290
Table III.2 Variation of segregation distance with transport index
for a single group of packages stowed below main deck
on a passenger aircraft . . . . . . . . . . . . . . . . . . . . . . . . . . 291
Table III.3 Variation of segregation distance with transport index
for main deck stowage on a combi or cargo aircraft . . . . 294
Table IV.1
Table V.1
Table V.2
Table V.3
Basic elements of quality assurance programmes that
should be considered and addressed in the safe transport
of radioactive material . . . . . . . . . . . . . . . . . . . . . . . . . . 300
Acceleration factors for package retention
system design . . . . . . . . . . . . . . . . . . . . . . . . . . . . . . . . . 318
Acceleration factors for package retention
system design for specific packages . . . . . . . . . . . . . . . . 319
Symbols used in calculation of a rectangular package
with baseplate flange bolted to the conveyance . . . . . . . . 325
Section I
INTRODUCTION
BACKGROUND
103.1. When making national or international shipments it is necessary to consult
the Regulations for the particular mode of transport to be used for the countries where
the shipment will be made. While most of the major requirements are in agreement
with the Regulations, there can be differences with respect to the assignment of
responsibilities for carrying out specific actions. For air shipments, the International
Civil Aviation Organization’s (ICAO) Technical Instructions for the Safe Transport of
Dangerous Goods by Air and the International Air Transport Association’s (IATA)
Dangerous Goods Regulations should be consulted, with particular regard to the State
and operator variations. For sea shipments, the International Maritime Organization’s
(IMO) International Maritime Dangerous Goods (IMDG) Code should be consulted.
Some countries have adopted the Regulations by reference while others have incorporated
them into their national regulations with possibly some minor variations.
OBJECTIVE
104.1. In general the Regulations aim to provide a uniform and adequate level of
safety that is commensurate with the inherent hazard presented by the radioactive
material being transported. To the extent feasible, safety features are required to be
built into the design of the package. By placing primary reliance on the package
design and preparation, the need for any special actions during carriage, i.e. by the
carrier, is minimized. Nevertheless, some operational controls are required for safety
purposes.
SCOPE
106.1. Transport includes carriage by a common carrier or by the owner or employee
where the carriage is incidental to the use of the radioactive materials, such as
vehicles carrying radiography devices being driven to and from the operations site by
the radiographer, vehicles carrying density measuring gauges being driven to and
from the construction site, and oil well logging vehicles carrying measuring devices
containing radioactive materials and radioactive materials used in oil well injections.
1
107.1. The Regulations are not intended to be applied to movements of radioactive
material that forms an integral part of a means of transport, such as depleted uranium
counterweights or tritium exit signs used in aircraft; or to radioactive material in persons
or animals for medical or veterinary purposes, such as cardiac pacemakers or
radioactive material introduced into humans or animals for diagnosis or treatment. The
treating physician or veterinarian should give appropriate advice on radiological safety.
107.2. Consumer products are items available to the general public as the end user
without further control or restriction. These may be devices such as smoke detectors,
luminous dials or ion generating tubes that contain small amounts of radioactive substances.
Consumer products are outside the scope of the Regulations only after sale
to the end user. Any transport, including the use of conveyances between manufacturers,
distributors and retailers, is within the scope of the Regulations to ensure that
large quantities of individually exempted consumer products are not transported in an
unregulated manner.
107.3. The principles of exemption and their application to the transport of radioactive
material are dealt with in para. 401.
107.4. The scope of the Regulations includes consideration of those natural materials
or ores which form part of the nuclear fuel cycle or which will be processed in
order to use their radioactive properties. The Regulations do not apply to other ores
which may contain naturally occurring radionuclides, but whose usefulness does not
lie in the fissile, fertile or radioactive properties of those nuclides, provided that the
activity concentration does not exceed 10 times the exempt activity concentration values.
In addition, the Regulations do not apply to natural materials and ores containing
naturally occurring radionuclides which have been processed (up to 10 times the
exempt activity concentration values) where the physical and/or chemical processing
was not for the purpose of extracting radionuclides, e.g. washed sands and tailings
from alumina refining. Were this not the case, the Regulations would have to be
applied to enormous quantities of material that present a very low hazard. However,
there are ores in nature where the activity concentration is much higher than the
exemption values. The regular transport of these ores may require consideration of
radiation protection measures. Hence, a factor of 10 times the exemption values for
activity concentration was chosen as providing an appropriate balance between the
radiological protection concerns and the practical inconvenience of regulating large
quantities of material with low activity concentrations of naturally occurring radionuclides.
108.1. Although these Regulations provide for the requisite safety in transport
without the need for specified routing, the regulatory authorities in some Member
2
Data Management, Analytics and Innovation: Proceedings of ICDMAI 2019, Volume 1 [1st ed. 2020] 978-981-32-9948-1, 978-981-32-9949-8
Table of contents :
Front Matter ....Pages i-xiv
Front Matter ....Pages 1-1
Empirical Study of Soft Clustering Technique for Determining Click Through Rate in Online Advertising (Akshi Kumar, Anand Nayyar, Shubhangi Upasani, Arushi Arora)....Pages 3-13
ASK Approach: A Pre-migration Approach for Legacy Application Migration to Cloud (Sanjeev Kumar Yadav, Akhil Khare, Choudhary Kavita)....Pages 15-27
A Fuzzy Logic Based Cardiovascular Disease Risk Level Prediction System in Correlation to Diabetes and Smoking (Kanak Saxena, Umesh Banodha)....Pages 29-40
An Integrated Fault Classification Approach for Microgrid System (Ruchita Nale, Ruchi Chandrakar, Monalisa Biswal)....Pages 41-56
Role of Data Analytics in Human Resource Management for Prediction of Attrition Using Job Satisfaction (Neerja Aswale, Kavya Mukul)....Pages 57-67
A Study of Business Performance Management in Special Reference to Automobile Industry (Gurinder Singh, Smiti Kashyap, Kanika Singh Tomar, Vikas Garg)....Pages 69-92
Secure Online Voting System Using Biometric and Blockchain (Dipti Pawade, Avani Sakhapara, Aishwarya Badgujar, Divya Adepu, Melvita Andrade)....Pages 93-110
An Approach: Applicability of Existing Heterogeneous Multicore Real-Time Task Scheduling in Commercially Available Heterogeneous Multicore Systems (Kalyan Baital, Amlan Chakrabarti)....Pages 111-124
Analyzing the Detectability of Harmful Postures for Patient with Hip Prosthesis Based on a Single Accelerometer in Mobile Phone (Kitti Naonueng, Opas Chutatape, Rong Phoophuangpairoj)....Pages 125-138
Software Development Process Evolution in Malaysian Companies (Rehan Akbar, Asif Riaz Khan, Kiran Adnan)....Pages 139-150
Automated Scheduling of Hostel Room Allocation Using Genetic Algorithm (Rayner Alfred, Hin Fuk Yu)....Pages 151-160
Evaluation of ASTER TIR Data-Based Lithological Indices in Parts of Madhya Pradesh and Chhattisgarh State, India (Himanshu Govil, Subhanil Guha, Prabhat Diwan, Neetu Gill, Anindita Dey)....Pages 161-169
Analyzing Linear Relationships of LST with NDVI and MNDISI Using Various Resolution Levels of Landsat 8 OLI and TIRS Data (Himanshu Govil, Subhanil Guha, Prabhat Diwan, Neetu Gill, Anindita Dey)....Pages 171-184
Automatic Robot Processing Using Speech Recognition System (S. Elavarasi, G. Suseendran)....Pages 185-195
Banking and FinTech (Financial Technology) Embraced with IoT Device (G. Suseendran, E. Chandrasekaran, D. Akila, A. Sasi Kumar)....Pages 197-211
Front Matter ....Pages 213-213
GRNN++: A Parallel and Distributed Version of GRNN Under Apache Spark for Big Data Regression (Sk. Kamaruddin, Vadlamani Ravi)....Pages 215-227
An Entropy-Based Technique for Conferences Ranking (Fiaz Majeed, Rana Azhar Ul Haq)....Pages 229-239
MapReduce mRMR: Random Forests-Based Email Spam Classification in Distributed Environment (V. Sri Vinitha, D. Karthika Renuka)....Pages 241-253
The Impact of Sustainable Development Report Disclosure on Tax Planning in Thailand (Sathaya Thanjunpong, Thatphong Awirothananon)....Pages 255-268
Clustering and Labeling Auction Fraud Data (Ahmad Alzahrani, Samira Sadaoui)....Pages 269-283
Big Data Security Challenges and Preventive Solutions (Nirmal Kumar Gupta, Mukesh Kumar Rohil)....Pages 285-299
Role and Challenges of Unstructured Big Data in Healthcare (Kiran Adnan, Rehan Akbar, Siak Wang Khor, Adnan Bin Amanat Ali)....Pages 301-323
Zip Zap Data—A Framework for ‘Personal Data Preservation’ (K. Arunkumar, A. Devendran)....Pages 325-337
A Systematic Mapping Study of Cloud Large-Scale Foundation—Big Data, IoT, and Real-Time Analytics (Isaac Odun-Ayo, Rowland Goddy-Worlu, Temidayo Abayomi-Zannu, Emanuel Grant)....Pages 339-363
Studies on Radar Imageries of Thundercloud by Image Processing Technique (Sonia Bhattacharya, Himadri Bhattacharyya Chakrabarty)....Pages 365-380
Front Matter ....Pages 381-381
PURAN: Word Prediction System for Punjabi Language News (Gurjot Singh Mahi, Amandeep Verma)....Pages 383-400
Implementation of hDE-HTS Optimized T2FPID Controller in Solar-Thermal System (Binod Shaw, Jyoti Ranjan Nayak, Rajkumar Sahu)....Pages 401-412
Design of Sigma-Delta Converter Using 65 nm CMOS Technology for Nerves Organization in Brain Machine Interface (Anil Kumar Sahu, G. R. Sinha, Sapna Soni)....Pages 413-423
Performance Comparison of Machine Learning Techniques for Epilepsy Classification and Detection in EEG Signal (Rekh Ram Janghel, Archana Verma, Yogesh Kumar Rathore)....Pages 425-438
Novel Approach for Plant Disease Detection Based on Textural Feature Analysis (Varinderjit Kaur, Ashish Oberoi)....Pages 439-449
Novel Approach for Brain Tumor Detection Based on Naïve Bayes Classification (Gurkarandesh Kaur, Ashish Oberoi)....Pages 451-462
Automatic Classification of Carnatic Music Instruments Using MFCC and LPC (Surendra Shetty, Sarika Hegde)....Pages 463-474
Semiautomated Ontology Learning to Provide Domain-Specific Knowledge Search in Marathi Language (Neelam Chandolikar, Pushkar Joglekar, Shivjeet Bhosale, Dipali Peddawad, Rajesh Jalnekar, Swati Shilaskar)....Pages 475-488
Identifying Influential Users on Social Network: An Insight (Ragini Krishna, C. M. Prashanth)....Pages 489-502
Factex: A Practical Approach to Crime Detection (Rachna Jain, Anand Nayyar, Shivam Bachhety)....Pages 503-516
Analysis of Classification Algorithms for Breast Cancer Prediction (S. P. Rajamohana, K. Umamaheswari, K. Karunya, R. Deepika)....Pages 517-528
Real-Time Footfall Prediction Using Weather Data: A Case on Retail Analytics (Garima Makkar)....Pages 529-542
Normal Pressure Hydrocephalus Detection Using Active Contour Coupled Ensemble Based Classifier (Pallavi Saha, Sankhadeep Chatterjee, Santanu Roy, Soumya Sen)....Pages 543-553
Question–Answer System on Episodic Data Using Recurrent Neural Networks (RNN) (Vineet Yadav, Vishnu Bharadwaj, Alok Bhatt, Ayush Rawal)....Pages 555-568
Convoluted Cosmos: Classifying Galaxy Images Using Deep Learning (Diganta Misra, Sachi Nandan Mohanty, Mohit Agarwal, Suneet K. Gupta)....Pages 569-579
Front Matter ....Pages 581-581
Energy-Based Improved MPR Selection in OLSR Routing Protocol (Rachna Jain, Indu Kashyap)....Pages 583-599
A Novel Approach for Better QoS in Cognitive Radio Ad Hoc Networks Using Cat Optimization (Lolita Singh, Nitul Dutta)....Pages 601-612
(T-ToCODE): A Framework for Trendy Topic Detection and Community Detection for Information Diffusion in Social Network (Reena Pagare, Akhil Khare, Shankar Chaudhary)....Pages 613-632
ns-3 Implementation of Network Mobility Basic Support (NEMO-BS) Protocol for Intelligent Transportation Systems (Prasanta Mandal, Manoj Kumar Rana, Punyasha Chatterjee, Arpita Debnath)....Pages 633-642
Modified DFA Minimization with Artificial Bee Colony Optimization in Vehicular Routing Problem with Time Windows (G. Niranjani, K. Umamaheswari)....Pages 643-662
Coverage-Aware Recharge Scheduling Scheme for Wireless Charging Vehicles in the Wireless Rechargeable Sensor Networks (Govind P. Gupta, Vrajesh Kumar Chawra)....Pages 663-671
A Transition Model from Web of Things to Speech of Intelligent Things in a Smart Education System (Ambrose A. Azeta, Victor I. Azeta, Sanjay Misra, M. Ananya)....Pages 673-683
Intrusion Detection and Prevention Systems: An Updated Review (Nureni Ayofe Azeez, Taiwo Mayowa Bada, Sanjay Misra, Adewole Adewumi, Charles Van der Vyver, Ravin Ahuja)....Pages 685-696
Simulation-Based Performance Analysis of Location-Based Opportunistic Routing Protocols in Underwater Sensor Networks Having Communication Voids (Sonali John, Varun G. Menon, Anand Nayyar)....Pages 697-711
A Hybrid Optimization Algorithm for Pathfinding in Grid Environment (B. Booba, A. Prema, R. Renugadevi)....Pages 713-721
Dynamic Hashtag Interactions and Recommendations: An Implementation Using Apache Spark Streaming and GraphX (Sonam Sharma)....Pages 723-738
Back Matter ....Pages 739-740
Citation preview
Advances in Intelligent Systems and Computing 1042
Neha Sharma Amlan Chakrabarti Valentina Emilia Balas Editors
Data Management, Analytics and Innovation Proceedings of ICDMAI 2019, Volume 1
Advances in Intelligent Systems and Computing Volume 1042
Series Editor Janusz Kacprzyk, Systems Research Institute, Polish Academy of Sciences, Warsaw, Poland Advisory Editors Nikhil R. Pal, Indian Statistical Institute, Kolkata, India Rafael Bello Perez, Faculty of Mathematics, Physics and Computing, Universidad Central de Las Villas, Santa Clara, Cuba Emilio S. Corchado, University of Salamanca, Salamanca, Spain Hani Hagras, School of Computer Science and Electronic Engineering, University of Essex, Colchester, UK László T. Kóczy, Department of Automation, Széchenyi István University, Gyor, Hungary Vladik Kreinovich, Department of Computer Science, University of Texas at El Paso, El Paso, TX, USA Chin-Teng Lin, Department of Electrical Engineering, National Chiao Tung University, Hsinchu, Taiwan Jie Lu, Faculty of Engineering and Information Technology, University of Technology Sydney, Sydney, NSW, Australia Patricia Melin, Graduate Program of Computer Science, Tijuana Institute of Technology, Tijuana, Mexico Nadia Nedjah, Department of Electronics Engineering, University of Rio de Janeiro, Rio de Janeiro, Brazil Ngoc Thanh Nguyen , Faculty of Computer Science and Management, Wrocław University of Technology, Wrocław, Poland Jun Wang, Department of Mechanical and Automation Engineering, The Chinese University of Hong Kong, Shatin, Hong Kong
The series “Advances in Intelligent Systems and Computing” contains publications on theory, applications, and design methods of Intelligent Systems and Intelligent Computing. Virtually all disciplines such as engineering, natural sciences, computer and information science, ICT, economics, business, e-commerce, environment, healthcare, life science are covered. The list of topics spans all the areas of modern intelligent systems and computing such as: computational intelligence, soft computing including neural networks, fuzzy systems, evolutionary computing and the fusion of these paradigms, social intelligence, ambient intelligence, computational neuroscience, artificial life, virtual worlds and society, cognitive science and systems, Perception and Vision, DNA and immune based systems, self-organizing and adaptive systems, e-Learning and teaching, human-centered and human-centric computing, recommender systems, intelligent control, robotics and mechatronics including human-machine teaming, knowledge-based paradigms, learning paradigms, machine ethics, intelligent data analysis, knowledge management, intelligent agents, intelligent decision making and support, intelligent network security, trust management, interactive entertainment, Web intelligence and multimedia. The publications within “Advances in Intelligent Systems and Computing” are primarily proceedings of important conferences, symposia and congresses. They cover significant recent developments in the field, both of a foundational and applicable character. An important characteristic feature of the series is the short publication time and world-wide distribution. This permits a rapid and broad dissemination of research results. ** Indexing: The books of this series are submitted to ISI Proceedings, EI-Compendex, DBLP, SCOPUS, Google Scholar and Springerlink **
More information about this series at http://www.springer.com/series/11156
Neha Sharma Amlan Chakrabarti Valentina Emilia Balas •
•
Editors
Data Management, Analytics and Innovation Proceedings of ICDMAI 2019, Volume 1
123
Editors Neha Sharma Society for Data Science Pune, Maharashtra, India Valentina Emilia Balas Department of Automatics and Applied Software Aurel Vlaicu University of Arad Arad, Romania
Amlan Chakrabarti A.K. Choudhury School of Information Technology University of Calcutta Kolkata, West Bengal, India
ISSN 2194-5357 ISSN 2194-5365 (electronic) Advances in Intelligent Systems and Computing ISBN 978-981-32-9948-1 ISBN 978-981-32-9949-8 (eBook) https://doi.org/10.1007/978-981-32-9949-8 © Springer Nature Singapore Pte Ltd. 2020 This work is subject to copyright. All rights are reserved by the Publisher, whether the whole or part of the material is concerned, specifically the rights of translation, reprinting, reuse of illustrations, recitation, broadcasting, reproduction on microfilms or in any other physical way, and transmission or information storage and retrieval, electronic adaptation, computer software, or by similar or dissimilar methodology now known or hereafter developed. The use of general descriptive names, registered names, trademarks, service marks, etc. in this publication does not imply, even in the absence of a specific statement, that such names are exempt from the relevant protective laws and regulations and therefore free for general use. The publisher, the authors and the editors are safe to assume that the advice and information in this book are believed to be true and accurate at the date of publication. Neither the publisher nor the authors or the editors give a warranty, expressed or implied, with respect to the material contained herein or for any errors or omissions that may have been made. The publisher remains neutral with regard to jurisdictional claims in published maps and institutional affiliations. This Springer imprint is published by the registered company Springer Nature Singapore Pte Ltd. The registered company address is: 152 Beach Road, #21-01/04 Gateway East, Singapore 189721, Singapore
Preface
These two volumes constitute the proceedings of the International Conference on Data Management, Analytics and Innovation (ICDMAI 2019) held from 18 to 20 January 2019. ICDMAI is a flagship conference of Society for Data Science, which is a non-profit professional association established to create a collaborative platform for bringing together technical experts across industry, academia, government laboratories and professional bodies to promote innovation around data science. ICDMAI 2019 envisions its role towards data science and its enhancement through collaboration, innovative methodologies and connections throughout the globe. The conference was hosted by Lincoln University College, Kuala Lumpur, Malaysia, and was supported by the industry leaders like IBM. Other partners for the conference were Wizer and DSMS College of Tourism & Management, West Bengal, India. The conference witnessed participants from 20 countries, 12 industries, 31 international universities and 94 premier Indian universities. Utmost care was taken in each and every facet of the conference, especially regarding the quality of the paper submissions. Out of 418 papers submitted to ICDMAI 2019, only 20% (87 papers) are selected for an oral presentation after a rigorous review process. Besides paper presentation, the conference also showcased workshops, tutorial talks, keynote sessions and plenary talks by the experts of the respective field. The volumes cover a broad spectrum of computer science, information technology, computational engineering, electronics and telecommunication, electrical, computer application and all the relevant disciplines. The conference papers included in these proceedings are published post-conference and are grouped into the four areas of research such as data management and smart informatics; big data management; artificial intelligence and data analytics; and advances in network technologies. All the four tracks of the conference were very relevant to the current technological advancements and had Best Paper Award in each track. Very stringent selection process was adopted for paper selection, and from plagiarism check to technical chairs’ review to double-blind review, every step was religiously followed. We compliment all the authors for submitting high-quality research papers to ICDMAI 2019. We would like to acknowledge all the authors for their contributions and also the efforts taken by reviewers and session chairs of the conference, v
vi
Preface
without whom it would have been difficult to select these papers. We appreciate the unconditional support from the members of the National and International Program Committee. It was really interesting to hear the participants of the conference highlight the new areas and the resulting challenges as well as opportunities. This conference has served as a vehicle for a spirited debate and discussion on many challenges that the world faces today. We especially thank our General Chair, Dr. P. K. Sinha; Vice Chancellor and Director, Dr. S. P. Mukherjee, International Institute of Information Technology, Naya Raipur (IIIT-NR), Chhattisgarh; other eminent personalities like Mr. Eddy Liew, Cloud and Solutions Country Technical Leader at IBM, Malaysia; Kranti Athalye, Sr. Manager in IBM India University Relations; Dr. Juergen Seitz, Head of Business Information Systems Department, Baden-Wuerttemberg Cooperative State University, Heidenheim, Germany; Mr. Aninda Bose, Senior Publishing Editor, Springer India Pvt. Ltd.; Dr. Vincenzo Piuri, IEEE Fellow, University of Milano, Italy; Hanaa Hachimi, National School of Applied Sciences ENSA in Kenitra, Morocco; Amol Dhondse, IBM Senior Solution Architect; Mohd Helmy Abd Wahab, Senior Lecturer and Former Head of Multimedia Engineering Lab at the Department of Computer Engineering, Faculty of Electrical and Electronic Engineering, Universiti Tun Hussein Onn Malaysia (UTHM); Anand Nayyar, Duy Tan University, Vietnam; and many more who were associated with ICDMAI 2019. Besides, there was CSI-Startup and Entrepreneurship Award to felicitate budding job creators. Our special thanks go to Janus Kacprzyk (Editor in Chief, Springer, Advances in Intelligent Systems and Computing Series) for the opportunity to organize this guest-edited volume. We are grateful to Springer, especially to Mr. Aninda Bose (Senior Publishing Editor, Springer India Pvt. Ltd.), for the excellent collaboration, patience and help during the evolvement of this volume. We are confident that the volumes will provide the state-of-the-art information to professors, researchers, practitioners and graduate students in the area of data management, analytics and innovation, and all will find this collection of papers inspiring and useful. Pune, India Kolkata, India Arad, Romania
Neha Sharma Amlan Chakrabarti Valentina Emilia Balas
Contents
Data Management and Smart Informatics Empirical Study of Soft Clustering Technique for Determining Click Through Rate in Online Advertising . . . . . . . . . . . . . . . . . . . . . . . . . . . Akshi Kumar, Anand Nayyar, Shubhangi Upasani and Arushi Arora
3
ASK Approach: A Pre-migration Approach for Legacy Application Migration to Cloud . . . . . . . . . . . . . . . . . . . . . . . . . . . . . . . . . . . . . . . . . Sanjeev Kumar Yadav, Akhil Khare and Choudhary Kavita
15
A Fuzzy Logic Based Cardiovascular Disease Risk Level Prediction System in Correlation to Diabetes and Smoking . . . . . . . . . . . . . . . . . . Kanak Saxena and Umesh Banodha
29
An Integrated Fault Classification Approach for Microgrid System . . . . Ruchita Nale, Ruchi Chandrakar and Monalisa Biswal
41
Role of Data Analytics in Human Resource Management for Prediction of Attrition Using Job Satisfaction . . . . . . . . . . . . . . . . . . Neerja Aswale and Kavya Mukul
57
A Study of Business Performance Management in Special Reference to Automobile Industry . . . . . . . . . . . . . . . . . . . . . . . . . . . . . . . . . . . . . Gurinder Singh, Smiti Kashyap, Kanika Singh Tomar and Vikas Garg
69
Secure Online Voting System Using Biometric and Blockchain . . . . . . . Dipti Pawade, Avani Sakhapara, Aishwarya Badgujar, Divya Adepu and Melvita Andrade
93
An Approach: Applicability of Existing Heterogeneous Multicore Real-Time Task Scheduling in Commercially Available Heterogeneous Multicore Systems . . . . . . . . . . . . . . . . . . . . . . . . . . . . . . . . . . . . . . . . . 111 Kalyan Baital and Amlan Chakrabarti
vii
viii
Contents
Analyzing the Detectability of Harmful Postures for Patient with Hip Prosthesis Based on a Single Accelerometer in Mobile Phone . . . . . . . . 125 Kitti Naonueng, Opas Chutatape and Rong Phoophuangpairoj Software Development Process Evolution in Malaysian Companies . . . . 139 Rehan Akbar, Asif Riaz Khan and Kiran Adnan Automated Scheduling of Hostel Room Allocation Using Genetic Algorithm . . . . . . . . . . . . . . . . . . . . . . . . . . . . . . . . . . . . . . . . . . . . . . . . 151 Rayner Alfred and Hin Fuk Yu Evaluation of ASTER TIR Data-Based Lithological Indices in Parts of Madhya Pradesh and Chhattisgarh State, India . . . . . . . . . . . . . . . . 161 Himanshu Govil, Subhanil Guha, Prabhat Diwan, Neetu Gill and Anindita Dey Analyzing Linear Relationships of LST with NDVI and MNDISI Using Various Resolution Levels of Landsat 8 OLI and TIRS Data . . . . . . . . . . . . . . . . . . . . . . . . . . . . . . . . . . . . . . . . . . . . 171 Himanshu Govil, Subhanil Guha, Prabhat Diwan, Neetu Gill and Anindita Dey Automatic Robot Processing Using Speech Recognition System . . . . . . . 185 S. Elavarasi and G. Suseendran Banking and FinTech (Financial Technology) Embraced with IoT Device . . . . . . . . . . . . . . . . . . . . . . . . . . . . . . . . . . . . . . . . . . . 197 G. Suseendran, E. Chandrasekaran, D. Akila and A. Sasi Kumar Big Data Management GRNN++: A Parallel and Distributed Version of GRNN Under Apache Spark for Big Data Regression . . . . . . . . . . . . . . . . . . . . 215 Sk. Kamaruddin and Vadlamani Ravi An Entropy-Based Technique for Conferences Ranking . . . . . . . . . . . . 229 Fiaz Majeed and Rana Azhar Ul Haq MapReduce mRMR: Random Forests-Based Email Spam Classification in Distributed Environment . . . . . . . . . . . . . . . . . . . . . . . 241 V. Sri Vinitha and D. Karthika Renuka The Impact of Sustainable Development Report Disclosure on Tax Planning in Thailand . . . . . . . . . . . . . . . . . . . . . . . . . . . . . . . . . . . . . . . 255 Sathaya Thanjunpong and Thatphong Awirothananon Clustering and Labeling Auction Fraud Data . . . . . . . . . . . . . . . . . . . . 269 Ahmad Alzahrani and Samira Sadaoui
Contents
ix
Big Data Security Challenges and Preventive Solutions . . . . . . . . . . . . . 285 Nirmal Kumar Gupta and Mukesh Kumar Rohil Role and Challenges of Unstructured Big Data in Healthcare . . . . . . . . 301 Kiran Adnan, Rehan Akbar, Siak Wang Khor and Adnan Bin Amanat Ali Zip Zap Data—A Framework for ‘Personal Data Preservation’ . . . . . . 325 K. Arunkumar and A. Devendran A Systematic Mapping Study of Cloud Large-Scale Foundation—Big Data, IoT, and Real-Time Analytics . . . . . . . . . . . . . . . . . . . . . . . . . . . . 339 Isaac Odun-Ayo, Rowland Goddy-Worlu, Temidayo Abayomi-Zannu and Emanuel Grant Studies on Radar Imageries of Thundercloud by Image Processing Technique . . . . . . . . . . . . . . . . . . . . . . . . . . . . . . . . . . . . . . . . . . . . . . . . 365 Sonia Bhattacharya and Himadri Bhattacharyya Chakrabarty Artificial Intelligence and Data Analysis PURAN: Word Prediction System for Punjabi Language News . . . . . . . 383 Gurjot Singh Mahi and Amandeep Verma Implementation of hDE-HTS Optimized T2FPID Controller in Solar-Thermal System . . . . . . . . . . . . . . . . . . . . . . . . . . . . . . . . . . . . 401 Binod Shaw, Jyoti Ranjan Nayak and Rajkumar Sahu Design of Sigma-Delta Converter Using 65 nm CMOS Technology for Nerves Organization in Brain Machine Interface . . . . . . . . . . . . . . . 413 Anil Kumar Sahu, G. R. Sinha and Sapna Soni Performance Comparison of Machine Learning Techniques for Epilepsy Classification and Detection in EEG Signal . . . . . . . . . . . . 425 Rekh Ram Janghel, Archana Verma and Yogesh Kumar Rathore Novel Approach for Plant Disease Detection Based on Textural Feature Analysis . . . . . . . . . . . . . . . . . . . . . . . . . . . . . . . . . . . . . . . . . . . 439 Varinderjit Kaur and Ashish Oberoi Novel Approach for Brain Tumor Detection Based on Naïve Bayes Classification . . . . . . . . . . . . . . . . . . . . . . . . . . . . . . . . . . . . . . . . 451 Gurkarandesh Kaur and Ashish Oberoi Automatic Classification of Carnatic Music Instruments Using MFCC and LPC . . . . . . . . . . . . . . . . . . . . . . . . . . . . . . . . . . . . . . 463 Surendra Shetty and Sarika Hegde
x
Contents
Semiautomated Ontology Learning to Provide Domain-Specific Knowledge Search in Marathi Language . . . . . . . . . . . . . . . . . . . . . . . . 475 Neelam Chandolikar, Pushkar Joglekar, Shivjeet Bhosale, Dipali Peddawad, Rajesh Jalnekar and Swati Shilaskar Identifying Influential Users on Social Network: An Insight . . . . . . . . . 489 Ragini Krishna and C. M. Prashanth Factex: A Practical Approach to Crime Detection . . . . . . . . . . . . . . . . . 503 Rachna Jain, Anand Nayyar and Shivam Bachhety Analysis of Classification Algorithms for Breast Cancer Prediction . . . . 517 S. P. Rajamohana, K. Umamaheswari, K. Karunya and R. Deepika Real-Time Footfall Prediction Using Weather Data: A Case on Retail Analytics . . . . . . . . . . . . . . . . . . . . . . . . . . . . . . . . . . . . . . . . . 529 Garima Makkar Normal Pressure Hydrocephalus Detection Using Active Contour Coupled Ensemble Based Classifier . . . . . . . . . . . . . . . . . . . . . . . . . . . . 543 Pallavi Saha, Sankhadeep Chatterjee, Santanu Roy and Soumya Sen Question–Answer System on Episodic Data Using Recurrent Neural Networks (RNN) . . . . . . . . . . . . . . . . . . . . . . . . . . . . . . . . . . . . . . . . . . . 555 Vineet Yadav, Vishnu Bharadwaj, Alok Bhatt and Ayush Rawal Convoluted Cosmos: Classifying Galaxy Images Using Deep Learning . . . . . . . . . . . . . . . . . . . . . . . . . . . . . . . . . . . . . . . . . . . . . . . . . 569 Diganta Misra, Sachi Nandan Mohanty, Mohit Agarwal and Suneet K. Gupta Advances in Network Technologies Energy-Based Improved MPR Selection in OLSR Routing Protocol . . . . . . . . . . . . . . . . . . . . . . . . . . . . . . . . . . . . . . . . . . . . . . . . . 583 Rachna Jain and Indu Kashyap A Novel Approach for Better QoS in Cognitive Radio Ad Hoc Networks Using Cat Optimization . . . . . . . . . . . . . . . . . . . . . . . . . . . . . 601 Lolita Singh and Nitul Dutta (T-ToCODE): A Framework for Trendy Topic Detection and Community Detection for Information Diffusion in Social Network . . . . . . . . . . . . . . . . . . . . . . . . . . . . . . . . . . . . . . . . . . 613 Reena Pagare, Akhil Khare and Shankar Chaudhary ns-3 Implementation of Network Mobility Basic Support (NEMO-BS) Protocol for Intelligent Transportation Systems . . . . . . . . . . . . . . . . . . . 633 Prasanta Mandal, Manoj Kumar Rana, Punyasha Chatterjee and Arpita Debnath
Contents
xi
Modified DFA Minimization with Artificial Bee Colony Optimization in Vehicular Routing Problem with Time Windows . . . . . . . . . . . . . . . . 643 G. Niranjani and K. Umamaheswari Coverage-Aware Recharge Scheduling Scheme for Wireless Charging Vehicles in the Wireless Rechargeable Sensor Networks . . . . . . . . . . . . 663 Govind P. Gupta and Vrajesh Kumar Chawra A Transition Model from Web of Things to Speech of Intelligent Things in a Smart Education System . . . . . . . . . . . . . . . . . . . . . . . . . . . 673 Ambrose A. Azeta, Victor I. Azeta, Sanjay Misra and M. Ananya Intrusion Detection and Prevention Systems: An Updated Review . . . . . 685 Nureni Ayofe Azeez, Taiwo Mayowa Bada, Sanjay Misra, Adewole Adewumi, Charles Van der Vyver and Ravin Ahuja Simulation-Based Performance Analysis of Location-Based Opportunistic Routing Protocols in Underwater Sensor Networks Having Communication Voids . . . . . . . . . . . . . . . . . . . . . . . . . . . . . . . . 697 Sonali John, Varun G. Menon and Anand Nayyar A Hybrid Optimization Algorithm for Pathfinding in Grid Environment . . . . . . . . . . . . . . . . . . . . . . . . . . . . . . . . . . . . . . . . . . . . . 713 B. Booba, A. Prema and R. Renugadevi Dynamic Hashtag Interactions and Recommendations: An Implementation Using Apache Spark Streaming and GraphX . . . . . 723 Sonam Sharma Author Index . . . . . . . . . . . . . . . . . . . . . . . . . . . . . . . . . . . . . . . . . . . . . . . . 739
About the Editors
Neha Sharma is the Founder Secretary of the Society for Data Science, India. She was the Director of the Zeal Institute of Business Administration, Computer Application & Research, Pune, Maharashtra, India, and and Deputy Director, of the Padmashree Dr. D.Y.Patil Institute of Master of Computer Applications, Akurdi, Pune. She completed her PhD at the prestigious Indian Institute of Technology (IIT-ISM), Dhanbad, and she is a Senior IEEE member as well as Execom member of IEEE Pune Section. She has published numerous research papers in respected international journals. She received the “Best PhD Thesis Award” and “Best Paper Presenter at International Conference Award” from the Computer Society of India. Her areas of interest include data mining, database design, analysis and design, artificial intelligence, big data, cloud computing, blockchain and data science. Amlan Chakrabarti currently the Dean of the Faculty of Engineering and Technology, Professor and Director of the A.K.Choudhury School of Information Technology, University of Calcutta, India. He was a postdoctoral fellow at the School of Engineering, Princeton University, USA from 2011 to 2012. He has published around 130 research papers in refereed journals and conferences, and has been involved in research projects supported by various national funding agencies and international collaborations. He is a senior member of the IEEE and ACM, ACM Distinguished Speaker, Vice President of the Society for Data Science, and Secretary of the xiii
xiv
About the Editors
IEEE CEDA India Chapter. He is also the Guest Editor of the Springer Nature Journal on Applied Sciences. His research interests include quantum computing, VLSI design, embedded system design, computer vision and analytics. Valentina Emilia Balas is currently a Full Professor at the Department of Automatics and Applied Software at the Faculty of Engineering, “Aurel Vlaicu” University of Arad, Romania. She holds a Ph.D. in Applied Electronics and Telecommunications from the Polytechnic University of Timisoara. Dr. Balas is the author of more than 300 research papers in refereed journals and international conferences. Her research interests include intelligent systems, fuzzy control, soft computing, smart sensors, information fusion, modeling and simulation. She is the editor‐in-chief of the International Journal of Advanced Intelligence Paradigms (IJAIP) and the International Journal of Computational Systems Engineering (IJCSysE), and is an editorial board member of several national and international journals.
Data Management and Smart Informatics
Empirical Study of Soft Clustering Technique for Determining Click Through Rate in Online Advertising Akshi Kumar, Anand Nayyar, Shubhangi Upasani and Arushi Arora
Abstract Online advertising is an industry with the potential for maximum revenue extraction. Displaying the ad which is more likely to be clicked plays a crucial role in generating maximum revenue. A high click through rate (CTR) is an indication that the user finds the ad useful and relevant. For suitable placement of ads online and rich user experience, determining CTR has become imperative. Accurate estimation of CTR helps in placement of advertisements in relevant locations which would result in more profits and return of investment for the advertisers and publishers. This paper presents the application of a soft clustering method namely fuzzy c-means (FCM) clustering for determining if a particular ad would be clicked by the user or not. This is done by classifying the ads in the dataset into broad clusters depending on whether they were actually clicked or not. This way the kind of advertisements that the user is interested in can be found out and subsequently more advertisements of the same kind can be recommended to him, thereby increasing the CTR of the displayed ads. Experimental results show that FCM outperforms k-means clustering (KMC) in determining CTR. Keywords Advertising
Click Through Rate Clustering
A. Kumar Department of Computer Science and Engineering, Delhi Technological University, Delhi, India e-mail: [email protected] A. Nayyar Graduate School, Duy Tan University, Da Nang, Viet Nam e-mail: [email protected] S. Upasani Department of Electronics and Communication Engineering, Delhi Technological University, Delhi, India e-mail: [email protected] A. Arora (&) Department of Electrical Engineering, Delhi Technological University, Delhi, India e-mail: [email protected] © Springer Nature Singapore Pte Ltd. 2020 N. Sharma et al. (eds.), Data Management, Analytics and Innovation, Advances in Intelligent Systems and Computing 1042, https://doi.org/10.1007/978-981-32-9949-8_1
3
4
A. Kumar et al.
1 Introduction Online advertising has become an important source of revenue for a wide range of businesses. With the tremendous growth in online advertising each year, it has also taken over a major area of research. Most of the revenues generated by widely used search engines as well as prevalent websites come from advertisements. It is therefore important to display relevant advertisements (ads) to the users and avoid the advertisements that are often disliked by them. Online advertising is more economical than traditional ways of advertising like mass markets and niche media. Internet ads have a wider audience and can be viewed for days and nights altogether, in contrast to ads on television and radios that last for shorter durations and are displayed with limited frequency. Market segmentation is much more effective over the Internet than in any other medium. Thorough study of markets, customer preferences and habits and segmenting consumers into cohesive groups can be done efficiently through online advertising. Online advertising also offers small businesses numerous benefits like robust targeting, consumer insights and more effective return on investment. Advertisements fall into two broad categories—sponsored search advertisements and contextual advertisements. Sponsored search ads are displayed on the same web pages that show results of search queries entered by users. The core purpose behind sponsored advertising is to enhance the advertiser’s brand image as the ads displayed have the same form and qualities as the advertiser’s original content. Contextual advertising, on the other hand, uses automated systems that display ads relevant to the user’s identity and website’s content. Google AdSense is one of the many well-known examples of contextual advertising. Google robots display only those ads that the users find relevant and useful. When a user visits a website, features like ad size, ad placement, etc. are extracted from the search query and sent to a server. Relevant ads are selected based on user’s past history, CTR and other data. Increasing the number of ads is not a good idea as it will shoot up the earnings only for a short time before the user switches to other search engines due to poor user experience. To maximize revenue, precise placements of ads are therefore required. CTR refers to the number of times the advertiser’s ad has been clicked (clicks) divided by number of times the ad appears on the screen (impression). Relevant placement of ad is a precondition for increasing the CTR for it. Ad performance can also be measured using CTR. CTR determination has several issues associated with it. The advertisers need to pay every time whenever an ad is clicked. It is on the basis of CTR that the search engine decides what ads are to be displayed and in what order of appearance. This is ensured by combining together the likelihood of an ad being clicked and the cost of the ad per click to create a display format that will yield maximum return. Many algorithms based on the supervised methodology have been designed to predict the CTR of advertisements like support vector machines, decision trees, Naïve Bayes, etc. Motivated by this, the goal is to analyse and assess the application of unsupervised approach namely FCM for determining the CTR of an advertisement. This
Empirical Study of Soft Clustering Technique for Determining …
5
clustering algorithm divides the total ads in the dataset into broad clusters based on whether they have been clicked or not by the user. This classification of ads helps in assessing the kind of advertisements that the user is really interested in and hence predict more of these kinds of ads. This would, in turn, result in a rise in the CTR of the displayed ads because of a greater number of clicks. The results obtained from FCM have been contrasted with KMC which has been used as a baseline model. The two techniques are assessed based on metrics like precision, recall and accuracy. The following content is compiled as follows: Sect. 2 reviews work done by various researchers along with the brief idea of the algorithm used by them and their results. Section 3 elaborates the dataset taken, methods employed for preparing the data before implementing the algorithm and the algorithms used. The scrutiny of the experimental outcomes is done in the Sect. 4. Section 5 culminates the results along with the suggestions for future research prospects.
2 Related Work After a thorough assessment of various studies in the past, it was found that a lot of algorithms have been proposed for the prediction of relevant ad to be displayed to the user. The author Avila Clemenshia et al. [1] in 2016, proposed a CTR prediction model using Poisson’s regression, linear regression and support vector regression algorithms and displayed the ads accordingly. The dataset was provided by a digital marketing agency. Their results stated that support vector regression performed best among the three. Evaluation of the results was done on the basis of root mean squared error (RMSE) and correlation coefficient. Authors Graepel et al. [2] in 2010 devised a new Bayesian CTR algorithm. The ad predictor presented showed better outcomes when compared to the baseline Naïve Bayes in terms of relative information gain (RIG) and areas under the curve (AUC). Hillard et al. [3] in 2010, implemented a model for estimating ad relevance. They refined it by including indirect feedback after consolidating the basic features of text overlap. In case of presence of adequate observations, click history was used. In case of no or few observations, a model was learned that could also be used for unpredicted ads. The precision, recall and f-score values were noted and improvement was observed with the new model. A logistic regression approach was suggested by author Kondakindi et al. [4] in 2014, to predict whether an ad will be clicked or not. The dataset used for this purpose was from Avazu provided as a part of Kaggle competition. They started off with simple Naive Bayes followed by Vowpal Wabbit and finally got the best scores with logistic regression together with proper data preprocessing. Shi et al. [5] in 2016, designed a framework for prediction of CTR and average cost per click (CPC) of a keyword using some machine learning algorithms. The performance data for the advertiser’s keywords was gathered from Google AdWords. The author had applied different machine learning algorithms such as regression, random forest
6
A. Kumar et al.
and gradient boosting to evaluate the prediction performance on both CTR and CPC. Results concluded that random forest transpires to be the best for both the metrics while gradient descent results are least reliable. Wang et al. [6] in 2011, implemented a model for learning of user’s click behaviours from advertisement search and click logs. Decision tree (DT), CRF, SVM and backpropagation neural networks (BPN) are the algorithms which were employed to carry out the imposition. The experimentation finally led to proving that CRF model outperformed the two baselines and SVM remarkably. Chakrabarti et al. [7] in 2008, developed a model for contextual advertising in which the revenue accrued by the site publisher and the advertising network depends upon the suitability of the ads displayed. This was followed by mapping the model to standard cosine similarity matching. Cheng et al. [8] in 2010, developed the model for customization of click models in sponsored search. The results demonstrated that the accuracy of CTR could significantly be improved by personalized models in sponsored advertising. Edizel* et al. [9] in 2017, proposed the use of that deep convolutional neural networks for CTR prediction of an advertisement. One approach involved query-ad depiction being learned at character while the second entail word-level model by pretrained word vectors. The conclusion signified better outcome than the standard machine learning algorithms trained with well-defined features. The analysis of the background work clearly connotes that most of the algorithm used in the past are supervised classification algorithms that involves two classes namely clicked and not clicked-demonstrating whether advertisement was clicked or not. The study of unsupervised clustering is still not much discovered in this domain to the extent of our understanding. This research paper is an endeavour to compare FCM and KMC algorithm using the dataset.
3 Data Characteristics The following Fig. 1 demonstrates the system architecture of the research. The following subsection explains the details.
3.1
Data Collection
The dataset is acquired from Avazu (Kaggle) for the purpose of writing this paper. It contains 11 days’ worth of data in order to build and test prediction models using various machine learning algorithms. As the given data is approximately 6 GB, the data taken was 10 h of data for training and 2 h of data for testing. The data is ordered chronologically and the clicks and non-clicks are sampled according to different strategies.
Empirical Study of Soft Clustering Technique for Determining …
Fig. 1 System architecture
The following features are included in the dataset: • id (unique id to identify advertisement) • click (0 for not clicked and 1 for clicked)
7
8
A. Kumar et al.
• hour (in format of YYMMDDHH; it refers to the date and time the data is recorded) • banner_pos (0 for top and 1 for bottom) • site_id (refers to the website id) • site_domain (website domain id) • site_category (category to which website belongs) • app_id (app id) • app_domain (app domain) • app_category (class to which app belongs) • device_id (id of device from which ad was clicked • device_ip (ip address of the device) • device_model (model number of device) • device_type (mobile/laptop/desktop) • device_conn_type(internet connection type-wifi/mobile data) • C1 and C14–C21 (anonymized categorical variables).
3.2
Preprocessing of Data
The class attribute is removed owing to the fact that unsupervised clustering is the technique applied which will learn from the data and classify it into two clusters. The dataset contains features in both integer and string format. In order to carry out the implementation, conversion of strings into integer is done. Pandas package in Python is used to load the csv file, i.e. the raw dataset into memory, identify the columns with string values and convert them into integer values using python standard hash function. Feature scaling is done with the help of scikit-learn library in python in the case of KMC. It is done in order to ensure fast convergence to the optimal solution and normalize the range of variables.
3.3
Implementation of Machine Learning Algorithms
The following section explains the clustering algorithm used. K-means Clustering: It is one of the most straightforward learning algorithms available for unsupervised learning [10]. The procedure basically follows two main steps to classify the dataset into k clusters. The number of clusters are fixed apriori. The algorithm starts by defining k cluster centers. Each value in the dataset is then associated to its nearest cluster. This is followed by a recalculation of the k cluster centers. A new binding is done between discrete units of information in the dataset and the new cluster centers and these steps are repeated till all units have been assigned to their respective clusters.
Empirical Study of Soft Clustering Technique for Determining …
9
The pseudocode is given as follows (Fig. 2): X = {x1, x2 …xn} be the collection of observations and u = {u1, u2 …uc} are the cluster centroids. We arbitrarily select ‘c’ cluster centers (1). Then, the distance
Fig. 2 Pseudocode for k-means clustering
10
A. Kumar et al.
between each observation in dataset and center is calculated. Following this, each observation is assigned to that cluster for which the distance between the observation and the center is least from all the available centers (2). This assignment step is followed by a recalculation of the cluster centers (3) by executing step 1 again. If all observations belong to the clusters calculated in the previous iteration, then the algorithm is terminated.
Fig. 3 Pseudocode for fuzzy c-means clustering
Empirical Study of Soft Clustering Technique for Determining …
11
Fuzzy C-Means Clustering: FCM is also referred to as soft clustering [11]. Each observation can be a part of more than one cluster at the same time. The distance between each cluster center and value in the dataset is evaluated and based on this distance, the degree of membership is assigned to each observation. A higher degree of membership towards a cluster means that the observation is closer to that cluster center than compared to the rest of the clusters. The summation of the degree of membership of each point in dataset equals to one. Data points belong to distinct clusters in hard or non-fuzzy clustering. The degree of membership assigned to each observation plays a pivotal role here. This denotes the extent to which an observation belongs to each cluster. As we move from center to the boundary of the cluster, the degree of belongingness of the observation decreases. The pseudocode for FCM is as follows (Fig. 3). Let X = {x1, x2, x3 …, xn} be the collection of discrete units in dataset and V = {c1, c2, c3 …, ck} be the centers. Select ‘k’ cluster centers and initialize the membership matrix in step (1). Compute the centers ‘cj’ in step (2). Calculate the membership ‘µij’ in the membership matrix using step (3). Repeat step (2) and (3) until the smallest value of J is obtained.
4 Results and Analysis The results were analysed using the accuracy, precision and recall [12] as an efficacy criterion. Accuracy is the measure of the closeness of the predicted observations to the actual value. It is the ratio of rightly predicted inspections to the total inspections made. Higher value of accuracy indicates more true positives and true negatives. Precision refers to the correctness of a model. It is simply the ratio of all the precisely predicted positives to the total number of positives predicted. More the number of true positives implies more precision. Recall is a measure of responsiveness or sensitivity of a machine model. It is the ratio of the correctly predicted positives to the count of values that belong to the class ‘yes’. Recall and precision are inversely related. The following Table 1 depicts the performance analysis of FCM and KMC. As Table 1 shows the accuracy, recall and precision values obtained for the two clusters, i.e. clicks/non-clicks predictions for the users is higher for FCM than for
Table 1 Comparison of results for k means and fuzzy c-means clustering
Accuracy Precision Recall
k-means Cluster 0
Cluster 1
Fuzzy c-means Cluster 0
Cluster 1
43.85438544 15.62280084 52.05182649
43.85438544 81.0428737 42.16809357
76.61767177 17.12049388 91.03165299
76.61767177 83.51293103 9.345230918
12
A. Kumar et al.
Fig. 4 Results
KMC. This means that the rightly predicted true positives and true negatives (accuracy), the degree of exactness (precision) and degree of completeness (recall) are relatively higher for FCM than for KMC. Both the algorithms divide the data into two clusters, Cluster 0 and Cluster 1, which in turn represent a division of the dataset into two categories (Fig. 4). These categories are based on click/non-click classification for the ads, i.e. one cluster has all the ads clicked by the user. The other cluster has all ads which were not clicked by him. This gives a clear idea of the type of ads the user is inquisitive about and willing to click on. After the classification of the ads in the dataset into categories—‘Click’ and ‘Non-Click’, we get a fair estimate of the kind of advertisements the user really wants to see. We can thereby predict more of such ads, hence increasing the CTR for the same. The number of ‘clicks’ divided by the total number of times the ad is displayed, i.e. ‘impressions’ gives the CTR.
5 Conclusion This paper compares the CTR as assessed by KMC and FCM algorithms. The outcome shows that FCM achieves better values of performance metrics than KMC. The results are better because it considers the fact that each data point can lie in more than one cluster and involves complex calculation of membership matrix. Conventional KMC, on the other hand, relies on hard clustering and definitively assigns each data point to the clusters. The accuracy of the model can further be improved by assessing the shape of the clusters, including other more refined attribute selection and extraction methods that could assist in better modelling of the present system. Attribute selection is
Empirical Study of Soft Clustering Technique for Determining …
13
concerned with selecting a subgroup of valid features for model selection whereas attribute extraction means deriving attributes from the already existing ones for subsequent learning. Superior results could be obtained by enhancing fuzziness coefficient. The degree of overlap between clusters is determined by the fuzziness coefficient. Higher value of m means larger overlapping between clusters. There exists a vast scope of application of other soft computing methodologies also, like swarm optimization, etc. that can be applied for determining CTR for other datasets as well.
References 1. Avila Clemenshia, P., Vijaya, M.S.: Click through rate prediction for display advertisement. Int. J. Comput. Appl. 975, 8887 (2016) 2. Graepel, T., Candela, J., Borchert, T., Herbrich, R.: Web-scale bayesian click-through rate prediction for sponsored search advertising in microsoft’s bing search engine. In: IJCA (2010) 3. Hillard, D., Schroedl, S., Manavoglu, E., Raghavan, H., Leggetter, C.: Im-proving ad relevance in sponsored search. In: ACM (2010) 4. Kondakindi, G., Rana, S., Rajkumar, A., Ponnekanti, S.K., Parakh, V.: A logistic regression approach to ad click prediction (2014) 5. Shi, L., Li, B.: Predict the click through rate and average cost per click for key-words using machine learning methodologies. In: IEOM (2016) 6. Wang., C.J., Chen, H.H.: Learning user behaviors for advertisements click predictions. In: ACM (2011) 7. Chakrabarti, D., Agarwal, D., Josifovski, V.: Contextual advertising by combining relevance with click feedback. In: WWW (2008) 8. Cheng, H., Cantú-Paz, E.: Personalized click prediction in sponsored search. In: ACM (2010) 9. Edizel, B., Mantrach, A., Bai, X.: Deep character-level click-through rate prediction for sponsored search. In: Stat.ml (2017) 10. Yadav, J., Sharma, M.: A review of K means algorithm. Int. J. Eng. Trends Technol. 4(7), 2972–2976 (2013) 11. Yanyun, C., Jianlin, Q., Xiang, G., Jianping, C., Dan, J., Li, C.: Advances in research of fuzzy c means algorithm. In: International Conference on Network Computing and Information Security (2011) 12. Bhatia, M.P.S., Kumar, A.: Information retrieval and machine learning: supporting technologies for web mining research and practice. Webology 5(2), 5 (2008)
ASK Approach: A Pre-migration Approach for Legacy Application Migration to Cloud Sanjeev Kumar Yadav, Akhil Khare and Choudhary Kavita
Abstract Legacy application migration is a mammoth task, if migration approach is not well thought at the very start, i.e. pre-migration, and supported by robust planning especially at pre-migration process area. This paper proposes a mathematical pre-migration approach, which will help the enterprise to analyse existing/ legacy application based on the application’s available information and parameters an enterprise would like to consider for analysis. Proposed pre-migration assessment will help in understanding the legacy application’s current state and will help in un-earthing the information with respect candidate application. Proposed pre-migration approach will help to take appropriate well-informed decision, whether to migrate or not to migrate the legacy application. As it is said that application migration is a journey, if kick-started once, needs to reach its destination else it can result into a disaster hence pre-migration is one of the important areas of migration journey.
Keywords Application migration ASK approach ASK confidence level (ACL) LAMP2C Legacy application Migration process Pre-migration
1 Introduction Technology paradigm is changing at fast pace, so is the customer requirement. In order to serve the fast-changing customer requirement is to keep abreast with ever-changing technology. Important is, if enterprise does not embrace or adopt S. K. Yadav (&) C. Kavita Jayoti Vidyapeeth Women’s University, Jaipur, India e-mail: [email protected] C. Kavita e-mail: [email protected] A. Khare CSED MVSR Engineering College, Hyderabad, India e-mail: [email protected] © Springer Nature Singapore Pte Ltd. 2020 N. Sharma et al. (eds.), Data Management, Analytics and Innovation, Advances in Intelligent Systems and Computing 1042, https://doi.org/10.1007/978-981-32-9949-8_2
15
16
S. K. Yadav et al.
new or up-coming technology then enterprise may face challenges such as unavailability or shortage of skilled manpower as they would have moved to other latest technology, which will impact software and hardware maintenance cost (complex patching and modifications/enhancement) because scarcity of people in market with the required technology will increase the manpower cost and at the same time even the hardware will also become costly. All this may put application scalability, business continuity, customers’ experience and enterprise image [1] in jeopardize and may result in inability to meet current business needs. Each of the challenges identified may have direct or indirect impact on one or more factors. At times these identified factors seem to be interrelated, refer Fig. 2. There may be other factors, which may be enterprise or legacy application specific. Enterprises may be ardent and ready to adopt technology such as cloud computing, which delivers resource as virtualize service [2] and offers so many other benefits. However, the biggest challenge for an organization is that enterprise can’t discard the investment made in the past in terms of existing business critical and in-use applications including its infrastructure. These business-critical applications/ systems are referred as an asset. These assets are built or might have evolved over a span of 10–20 years; and have become core to business process, might have become complex and large enough to be understood by a person. Cloud computing offers multiple benefits which any enterprise will be interested in such as lower operating cost, no up-front investment and ease of maintenance. Fact is that cloud reduces capital expenditure by leveraging cloud business model of pay-as-you use, which turns capital expenditure to operation expenditure. As exiting applications cannot be discarded, so to embrace the new technology enterprise has below-mentioned three options for lasting benefits/goal, refer Fig. 1:
P r o g r e s s
Migrate Development Phase
Maintenance Phase
Initial Phase
Time Fig. 1 Software life cycle phases and modernisation options©
Replace & Re-develop
ASK Approach: A Pre-migration Approach for Legacy Application …
i. Application platform. ii. Application available in iii. Application again.
17
Migration—Migrate legacy application migration to new Replacement—Replace legacy application with off-the-shelf, market, application. Redevelopment—Redevelop the existing application all over
Two options, Option ii, i.e. Application replacement or option iii, i.e. Application redevelopment, does not seem to be feasible options because the existing business-critical application might have got developed with lot of effort, time and cost. Putting similar effort, cost and time all over again may not be feasible, so feasible option available with enterprise is option i, i.e. Application migration, which will help to migrate current application to new technology/ platform and embrace new technology, i.e. Cloud at the same time, will keep the business-critical application intact. Paper is organized in six sections. Section 2 provides the literature review, which provides background of related work. Section 3 defines the problem. Section 4 provides the detail about the proposed framework. Section 5 details the ASK approach. Finally, the paper concludes with Sect. 6, which presents the concluding comments and future scope.
2 Literature Review Cloud computing acceptance has increased over a period of time because of the benefits it brings in the area of operational and efficiency. Migration frameworks are proposed and are compared in the selected literature but none provides an end-to-end migration framework, especially in the pre-migration stage of the migration. The focus of literature review is to bring out any available pre-migration method/approach. Summary of literature studied and identified research gap in context of chosen topic of the paper are detailed below: Shoaib et al. [3] highlighted that due to technology advancement enterprises need to migrate from one platform to other, so existed framework was examined and reviewed. Based on this, the author is of view that multiple methods exists for migration and at the same time there are multiple risks involved during migration all these risks can be taken care if migration is performed properly. In this paper, the author describes different methods and frameworks which provides guideline for developers to enhance software migration process. As per the author, the focus area for migration is framework along with process and activities. The author concludes that there is research gap and need to be explored further. Musale et al. [4] provided basic knowledge about the migration concept and initial factors required to migrate to cloud. The paper also highlighted that could computing is an emerging technology and there is substantial scope of operational
18
S. K. Yadav et al.
and efficiency improvement. If IT application is migration to cloud or some of the processes are migration to cloud environment, off premises. The author puts forward the cloud migration concept and discusses the essential points for migration and further discusses the after migration essential. The author is of view that migration to cloud is an art than it is a science. Gholami et al. [5] highlighted that research around migrating legacy application to cloud has increased because of the adoption of cloud computing as an outsourcing strategy has grown in recent past. The author is of view that there is no integrated overarching cloud migration exists in current literature. In order to fulfil this gap, the author tries to propose cloud platform-independent conceptual view and reusable migration process, which has phases, activities and task. Validity of the proposed metamodel is demonstrated by analysing three existing cloud migration process models. Model can be extended by adding new constructs and can be customized for other migration paradigm. Sabiri et al. [6] , the authors said that enterprises adopting cloud is increasing because of the significant advantage cloud computing brings in; however, the migration (move) to cloud has challenges. The authors studied and compared the existing cloud migration methods to understand the strengths and weaknesses of each model based on Model-Driven Engineering (MDE) approach. The author proposed and explained a cloud migration method based on Architecture Driven Modernization (ADM), which focuses on architectural modes instead of code artefacts. Gouda et al. [7] highlighted that cloud migration can be of two types; either from on-site premises to the cloud or moving them from one cloud environment to another, where the information can be accessed over the Internet. The authors also highlighted that cloud migration is broadly classified into two categories, i.e. Big Bang Migration and Trickle Migration. The authors have suggested multiple cloud migration patterns or categories as their requirement. The authors also suggested migration to cloud-step wise approach or procedure which will help the user to successful and fruitful migration of its IT resources to cloud. Shawky [2] highlighted that in order to utilize cloud’s capability, the system should be migrated to cloud. The author also highlights that application migration to cloud leads multiple technical challenges and these challenges cannot be ignored. According to the author, the main challenge is mapping application to cloud services as multiple aspects need to be considered, which includes other system component dependencies. Rashmi and Sahoo [8], ‘A five-phased approach for cloud migration’; highlighted that cloud computing is the rapidly growing segment of IT. Organization is moving to cloud due to its long-term benefits but they are unsure about the migration process. The authors proposed a five-phased waterfall-based migration model to cloud having feedback path to earlier phase of the process but have not dwelled into the details of each phase of the proposed model. Pahl et al. [9] mentioned in this paper that though cloud computing has gained the attention, adoption of the technology is yet to gain acceptance as how to migrate to cloud is unanswered for many. Point in case is highlighted with the help of three provider-driven case studies and tries to extract common layer-specific migration
ASK Approach: A Pre-migration Approach for Legacy Application …
19
process. Highlights that no common procedures and tools are exiting, however, establish migration core elements such as activities and steps. Stavru et al. [10] acknowledged the fact that enterprises are unable to take full strategic and operational advantage as they are not able to migrate legacy application to cloud due to non-existence of mature process. The authors identified and extracted challenges with the help of systematic literature review. Using expert judgment, evaluates how different agile techniques, taken from Scrum and Extreme Programming (XP), could address or overcome the identified challenges in software modernization projects in specific context.
3 Problem Specification Challenge can be in terms of skilled resource’s scarcity, software maintenance cost, lack of software scalability, business continuousness, customers experience, reduced efficiencies, knowledge retention, enterprise image [1] and inability to meet current business needs. Each of the challenges identified may have direct or indirect impact on one or more factors, so identified impacted factors seem to be interrelated. Tried categorizing these challenges into the below category; refer Fig. 2. There may be other factors, which may at times be enterprise specific or legacy application specific. • Customer (a) Application inefficient in catering to business requirement will impact the enterprise interest. (b) Applications may become inefficient in catering to any area of customer requirement (customer can be internal and/or external) over time; in terms of functionality/feature(s) and better service. Inefficient application will provide an edge to competition. • Knowledge (a) Lack of in-depth knowledge in terms of understanding the existing system and its related process will or may have direct impact on the system maintenance, i.e. developing new functionality and enhancements. Customer
Fig. 2 System maintenance and enhancement challenges
Technlogy
Compliance
Knowledge
Resource
20
S. K. Yadav et al.
(b) Due to non-availability of knowledgeable resources will have difficulty in getting best practices shared with respect to these legacy applications, i.e. knowledge sharing. It will increase the risk of knowledge concentration amount small set of people. • Resources (a) Reducing resource pool (attrition) or resource pool desire to move onto market relevant technology will have direct impact on resource availability for the maintenance/enhancement of legacy application. (b) As legacy application knowledge gets concentrated into few resources, it will lead to increase in people retaining cost; related aspect will be increase cost of gaining resource in desired technology. (c) Contracted resource pool will have risk of high dependency, in terms of understanding the existing system and its related process on to a very small set of people in an organization. • Technology (a) Enterprise may have multiple technologies in use for existing business-critical applications, so maintaining them will have high cost because the legacy application might have become unsupported due to non-existence of support from the product vendor as they might have stopped supporting the in-use version or vendor might release new version, i.e. vendor moved ahead in technology. • Compliance (a) Legacy applications may become non-regulatory compliance over a period of time if no enhancement is made due to multiple reasons mentioned above. (b) Non-compliance to regulatory norms may have bigger impact than adopting new technology and moving legacy application to the technology. Challenges highlighted above are few of the challenge, many other challenges will come which will be application or enterprise-specific challenges. These challenges motivate to come up with a framework, which will help the enterprise to migrate legacy application to cloud in an efficient manner.
4 Proposed Framework Legacy application migration is a journey which requires a careful detailed planning irrespective of the size of the application identified for migration because no one-size-fit-approach will work in application migration. One of the biggest challenges an enterprise faces is the uncertainty of where to begin the migration process. If enterprises do not carefully plan, execute and monitor the transformation using
ASK Approach: A Pre-migration Approach for Legacy Application …
21
established processes [11] then migration can be fraught with pitfalls. Before we proceed further, it is essential to understand the reasons or trigger points for migration. At high level there are only two factors which trigger migration and these factors are either: Business Driven, Technology Driven or both. Whichever may the reason for migration, i.e. business and/or technology; one thing which will happen first is current portfolio analysis. Cloud computing concepts and approach itself is very different from the traditional software concepts and approach. In fact, moving to cloud is a serious step to take in a holistic manner [1], which required a careful elaborate planning for successful migration because cloud computing brings changes such as economic, legal, privacy/security, [1] etc. especially the pre-migration area of the migration process. Cloud covers all application layers, i.e. application (SaaS), platform (PaaS) and infrastructure (IaaS), which makes legacy application migration not a straightforward task as it looks like. Rashmi et al. [8], especially when migrating a legacy application, which is tightly coupled [12] as compared to cloud application. In order to address this issue, a framework is proposed called ‘Legacy Application Migration Process Framework to Cloud’; referred as LAMP2C, Fig. 3. LAMP2C migration framework acts as a guiding principle for migrating application to cloud and process to be followed while migrating legacy application. Scope of the proposed LAMP2C framework covers entire legacy application as shown in Fig. 3 and pre-migration is highlighted as ASK Approach is part and limited to the pre-migration area of the LAMP2C framework. Framework helps to plan the migration in an effective and efficient manner and it also helps in speeding up migration execution process with clear and hazel free migration path. LAMP2C, address pre-migration, migration and post-migration area of migration with an umbrella of governance area across three cloud layers. Framework is divided into area and area is further sub-divided into phases. The phases will have activities under it. LAMP2C—high-level framework is shown in Fig. 4.
Fig. 3 High-level framework
22 Fig. 4 LAMP2C constituents
S. K. Yadav et al.
Activities Phases Area
LAMP2C is a flexible framework, which can be tweaked as per the enterprise’s requirement as proposed framework does not force to follow the process end-to-end rather it suggests to go phased manner. One of the important parts of LAMP2C is the pre-migration area, which helps in evaluating existing portfolio or application which in turn helps an enterprise in understanding the complexity, challenges and efforts that will be required for application migration. It will also address the uncertainty of ‘where to begin’ and other than whether to migrate or not to migrate to the cloud. LAMP2C, Figs. 1 and 3 is copyright of the authors: Registration Number L-60550/2014.
5 Ask Approach It is assumed that at high level there are only two factors which can trigger migration and they are either technical or non-technical (business) parameter, so assessment and its parameters are also clubbed into these two categories only. Another assumption is that a) technical assessment should consider all the three cloud’s service model, i.e. IaaS, SaaS and PaaS; however, enterprise is free to choose one or more for assessment and their respective parameters. Parameters which cut across three models should be grouped into one as ‘Common Parameters’, as shown in Fig. 5. Common parameters are to avoid the consideration of same parameter(s) across three layers of service model, however it will depend upon individual, how they would like to perform the pre-migration analysis. b) Non-technical, i.e. business parameter are those parameters, which are not technical in nature should be considered as one group or as suitable. ASK Approach—a pre-migration assessment methodology is proposed to perform portfolio or application analysis under pre-migration are of LAMP2C framework. ASK Approach is an important constituent of the LAMP2C framework and its scope is limited to the pre-migration area of the LAMP2C framework as it
ASK Approach: A Pre-migration Approach for Legacy Application …
23
Fig. 5 Parameter identification area
Identify Parameters
ASK Point
ASK Confidence Level
ASK Belt
Plot Graph
Fig. 6 Pre-migration approach
provides inputs and helps in taking GO/No-Go decision with respect to legacy application migration to cloud. Proposed pre-migration assessment methodology is parameter based mathematical approach, which will be appropriate for any application identified for migration and will help enterprise to view and analyse the enterprises IT application portfolio in details based on parameters which are important for organization and application so assessment is carried out on any set of identified parameters under the mentioned category or both, i.e. technical and non-technical (business) aspect. Pre-migration approach can be divided into five steps, as shown in Fig. 6 and detailed in subsequent paras: As approach is parameter based, as a first step, all parameters and its sub-parameters needs to be identified. Each identified and considered parameter needs to be assigned the importance. Importance is rated between the range of 0.1, being the lowest to 5.0, being the highest, based on the parameter’s impact, as tabled below (Table 1):
Table 1 Impact rating range
Importance
Impact rating range
Very high High Neutral Low Very low
4.1–5.0 3.1–4.0 2.1–3.0 1.1–2.0 0.1–1.0
24
S. K. Yadav et al.
Assuming that there are n number of parameters namely C1, C2, C3 and so on till Cn. Based on parameters’ importance an impact rating between the range of 0.1–5.0 will be assigned for each parameter. Adding all these integrals and dividing them by the total number of parameters (in this case n) considered for analysis will give us the mean value of the data, which is being referred as ‘ASK Point’ (ASKP), i.e. lc . R ASKP ¼ lc ¼
C1 þ
R
C2 þ
R
C3 þ n
R
C4 . . . þ
R
Cn
ASKP ¼ lc ¼ Parameters'Mean n ¼ Number of parameters i ¼ Importance of parameter In reduced form the above formula can be written as follows: n R X Cn ASKP ¼ lc ¼ n 1
Considering the same data calculate the standard, which will be referred as ASK Confidence Level (ACL). ACL will be calculated by adding the squares of the values found from subtracting the ASKP from each parametric value. This summed value is divided by the total number of parameters. This will give us the standard deviation rc , i.e. ACL. R 2 R 2 R 2 R 2 C1 lc þ C2 lc þ C3 lc þ Cn lc ACL ¼ rc ¼ n
ACL ¼ rc ¼ Parameters' Standard Deviation ASKP ¼ lc ¼ Parameters'Mean n ¼ Number of parameters In reduced form the above formula can be rewritten as follows: ACL ¼ rc ¼
n X 1
R
C n lc n
2
In order to generate boundary for the identified parameter, which is going to be referred as ASK Belt (ASKB). ASKP and ACL will help to build ASK Belt. ASKB will have two components a) ASKB Right, i.e. ASKBR and b) ASKB Left, i.e. ASKBL. ASKBR and ASKBL can be calculated as shown below: ASKBR ¼ ASKP þ ACL ASKBL ¼ ASKP ACL
ASK Approach: A Pre-migration Approach for Legacy Application …
25
Once, ASKP, ACL and ASKB (ASKBR and ASKBL); bell curve can be calculated using the below formula (Gaussian equation) ðxlc Þ2 1 f ð xÞ ¼ pffiffiffiffiffiffiffiffiffiffi e 2rc 2pr2c
The following are the value for the above formula: p = 3.14 e = 2.71 and x = Parameter Impact lc = ASKP rc = ACL As fifth and the last step; calculate various other values required in the Gaussian function. The parameters have been arranged in the ascending order of the impact rating for facilitating the plotting process. Plotting the above f ð xÞ values versus x values, we get the following bell curve (as defined by the Gaussian function), refer Fig. 7. Points which falls between ASKBR and ASKBL can be safely considered for legacy application migration, however, points which fall outside the belt need to be re-looked and considered with caution. These inputs will provide enough data points to conclude as to Go ahead with the legacy application migration or still there are points/parameters, which need to be re-looked at. And will provide inputs to come up with migration plan.
Fig. 7 Bell curve
26
S. K. Yadav et al.
Debian Bug report logs - #777439
Jessie DI-rc1 amd64 after installation no network interfaces
Reported by: Heiko Ernst <heiko.ernst@aschershain.de>
Date: Sun, 8 Feb 2015 09:27:01 UTC
Severity: important
Tags: bullseye, buster, d-i, jessie, sid, stretch
Merged with 694068, 727740
Found in version netcfg/1.102
Blocking fix for 696755: installation-reports: no network connection after reboot if only tasksel's standard and ssh-server
Reply or subscribe to this bug.
Toggle useless messages
View this report as an mbox folder, status mbox, maintainer mbox
Report forwarded to :
; Package . (Sun, 08 Feb 2015 09:27:05 GMT) (full text, mbox, link).
Acknowledgement sent to :
New Bug report received and forwarded. Copy sent to . (Sun, 08 Feb 2015 09:27:05 GMT) (full text, mbox, link).
Message #5 received at submit@bugs.debian.org (full text, mbox, reply):
Information forwarded to :
; Package . (Sun, 08 Feb 2015 09:42:04 GMT) (full text, mbox, link).
Acknowledgement sent to :
Extra info received and forwarded to list. Copy sent to . (Sun, 08 Feb 2015 09:42:04 GMT) (full text, mbox, link).
Message #10 received at 777439@bugs.debian.org (full text, mbox, reply):
Message sent on to :
Bug#777439. (Sun, 08 Feb 2015 09:42:08 GMT) (full text, mbox, link).
Information forwarded to :
; Package . (Sun, 08 Feb 2015 11:27:15 GMT) (full text, mbox, link).
Acknowledgement sent to :
Extra info received and forwarded to list. Copy sent to . (Sun, 08 Feb 2015 11:27:15 GMT) (full text, mbox, link).
Message #18 received at 777439@bugs.debian.org (full text, mbox, reply):
What’s New in the Sorenson Squeeze Compression Suite v4.2.301 6 serial key or number?
Screen Shot
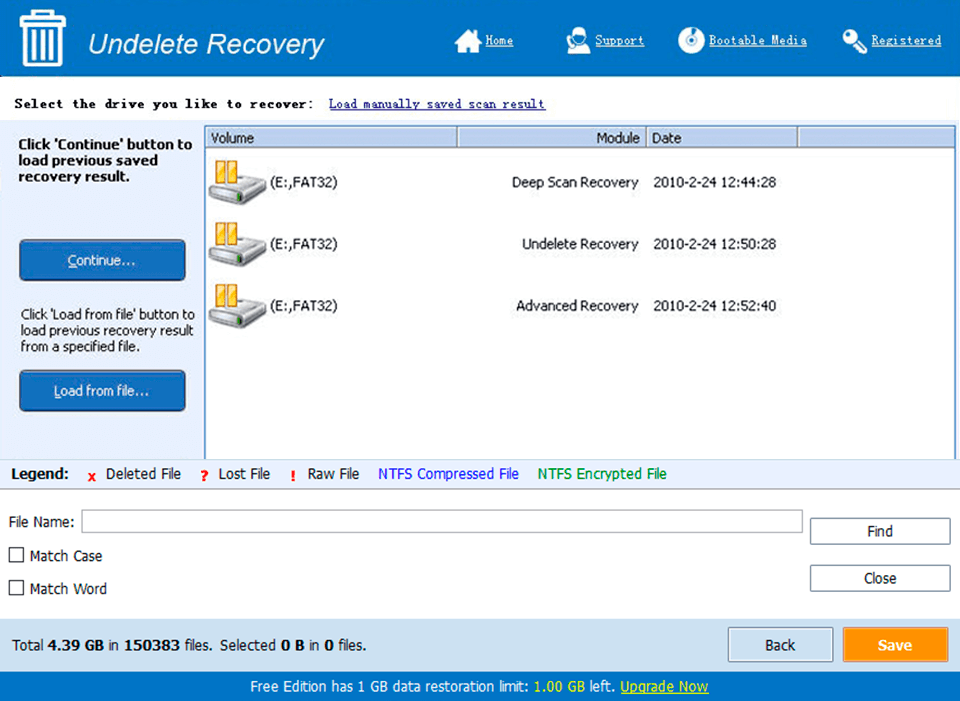
System Requirements for Sorenson Squeeze Compression Suite v4.2.301 6 serial key or number
- First, download the Sorenson Squeeze Compression Suite v4.2.301 6 serial key or number
-
You can download its setup from given links: