
Accurate ID Standard v2.5 serial key or number

Accurate ID Standard v2.5 serial key or number
CUTseq is a versatile method for preparing multiplexed DNA sequencing libraries from low-input samples
Abstract
Current multiplexing strategies for massively parallel sequencing of genomic DNA mainly rely on library indexing in the final steps of library preparation. This procedure is costly and time-consuming, because a library must be generated separately for each sample. Furthermore, library preparation is challenging in the case of fixed samples, such as DNA extracted from formalin-fixed paraffin-embedded (FFPE) tissues. Here we describe CUTseq, a method that uses restriction enzymes and in vitro transcription to barcode and amplify genomic DNA prior to library construction. We thoroughly assess the sensitivity and reproducibility of CUTseq in both cell lines and FFPE samples, and demonstrate an application of CUTseq for multi-region DNA copy number profiling within single FFPE tumor sections, to assess intratumor genetic heterogeneity at high spatial resolution. In conclusion, CUTseq is a versatile and cost-effective method for library preparation for reduced representation genome sequencing, which can find numerous applications in research and diagnostics.
Introduction
In the past decade, next-generation sequencing (NGS) technologies have become widely available in diagnostics and research laboratories1,2. During this time, the number of methodologies for preparing DNA libraries for NGS has greatly expanded, whereas the cost of sequencing has exponentially dropped1,2. In spite of this progress, sequencing of multiple samples in parallel remains costly, mainly due to the way in which multiplexing is achieved. Typically, this is done by indexing libraries prepared from individual samples, followed by pooling together multiple libraries in the same sequencing run. This means that all the steps in the library preparation procedure must be repeated for every sample to be sequenced, which is labor-intensive and multiplies the cost of reagents. Furthermore, accurate normalization of library concentration is necessary before multiple libraries can be pooled together, which is not always possible and requires additional reagents. In contrast, being able to directly barcode genomic DNA (gDNA) prior to library construction, followed by pooling of differentially barcoded samples into a single library, should enable high levels of multiplexing at much lower cost.
An example of application that would greatly benefit from improved solutions for NGS library multiplexing is multi-region DNA sequencing of tumor samples3. In this approach, DNA is extracted from multiple regions within the same tumor mass, or from multiple tumor sites in the same patient, and a library is prepared for each region. Multi-region tumor sequencing has been successfully used to assess levels of intratumor heterogeneity and to infer tumor evolution in different cancer types3. One limitation of current multi-region tumor sequencing approaches is the size of the regions examined, which must be sufficiently large to enable the recovery of enough DNA to construct a library from every region separately. This precludes the possibility of examining a larger number of smaller regions, e.g., within a single tissue section, which would enable assessing intratumor heterogeneity at much higher spatial resolution. This, together with the high cost needed to make a single library for every region sampled, currently limits the applicability of multi-region tumor sequencing in routine cancer diagnostics.
Several approaches have been developed to barcode gDNA as well as to amplify sub-nanogram amounts of gDNA prior to library preparation. Direct incorporation of sequencing adapters into gDNA by engineered transposases allows rapid library preparation and is the basis of successful commercial solutions such as Nextera from Illumina, Inc. However, this approach still requires that individual libraries are generated from each sample, and then pooled together before sequencing. On the other hand, whole-genome amplification methods, such as DOP-PCR4, MDA5, MALBAC6, and the more recent SCMDA7 and LIANTI8, achieve direct gDNA barcoding during genome amplification, so that multiple samples can be pooled together into a single multiplexed library. Although such methods are specifically tailored for whole-genome sequencing of single cells, they could, in principle, also be used for other applications, for instance to generate multiplexed libraries for multi-region tumor sequencing in tissue sections. One limitation, however, is that whole-genome amplification requires intact DNA and thus is problematic in fixed tissue samples, in particular formalin-fixed, paraffin-embedded (FFPE) specimens, which still represent a cornerstone in pathology. In addition, whole-genome amplification methods are very costly, making them hardly applicable to routine diagnostics.
To overcome these limitations, here we develop a method, which we name CUTseq, that combines restriction endonucleases with in vitro transcription (IVT), to construct highly multiplexed DNA libraries for reduced representation genome sequencing of multiple samples in parallel. We show that CUTseq can be used to barcode gDNA extracted from both non-fixed and fixed samples, including old archival FFPE tissue sections. We benchmark CUTseq by comparing it with a widely used method of DNA library preparation and demonstrate that CUTseq can be used for reduced representation genome and exome sequencing, enabling reproducible DNA copy number profiling and single-nucleotide variant (SNV) calling in both cell and low-input FFPE tissue samples. We then show an application of CUTseq for assessing intratumor genetic heterogeneity, by profiling DNA copy number levels in multiple small regions of individual FFPE tumor sections. Lastly, we describe a workflow for rapid and cost-effective preparation of highly multiplexed CUTseq libraries, which can be applied in the context of high-throughput genetic screens and for cell line authentication.
Results
CUTseq workflow
We aimed at developing a versatile method for preparing highly multiplexed DNA sequencing libraries, by barcoding gDNA from multiple samples directly after purification. To this end, we devised the CUTseq workflow as depicted in Fig. 1a. The procedure starts by digesting gDNA extracted from either non-fixed or fixed samples, including low-input FFPE tissue specimens, using a type-II restriction endonuclease that leaves staggered ends. After gDNA is digested, the restricted sites are ligated to specialized double-stranded DNA adapters that contain a sample-specific barcode sequence, a unique molecular identifier (UMI)9, the RA5 Illumina sequencing adapter, and the T7 promoter sequence. After ligation, multiple samples are pooled together and the genomic sequences flanking the ligated restriction sites are amplified using IVT by the T7 RNA polymerase. Lastly, a sequencing library is generated from the IVT product, based on the small RNA library preparation kit from Illumina (Methods). A step-by-step CUTseq protocol is available in the Supplementary Methods and at Protocol Exchange (https://doi.org/10.21203/rs.2.1742/v1). The sequences of all the CUTseq adapters used in this study are provided in Supplementary Data 1.
CUTseq implementation
We first tested the feasibility of CUTseq by constructing libraries from gDNA extracted from five different human cancer cell lines and IMR90 primary human fibroblasts (Methods). We digested the samples using either a more frequent four-base cutter (NlaIII) or a less frequent six-base cutter (HindIII). We selected the enzymes among a list of commercially available restriction enzymes that leave staggered DNA ends and are methylation insensitive (Supplementary Table 1), choosing the least expensive enzymes with the most homogeneous distribution of recognition sites in the human genome (Supplementary Fig. 1a-d). The distance between two consecutive recognition sites is 210 ± 286 bp and 3422 ± 3684 bp (mean ± SD) for NlaIII and HindIII, respectively. We sequenced all the libraries on the NextSeq 500 platform from Illumina, Inc., and processed the reads through a custom-made pipeline that we make freely available (Supplementary Software). All the libraries showed a homogeneous fragment size distribution and yielded a high proportion of reads with the expected prefix (95% ± 0.01%, mean ± SD), high mappability (96.58% ± 0.02%, mean ± SD), very low rate of sequencing errors (0.81% ± 0.001%, mean ± SD), even partitioning between the Watson and Crick strands, and balanced distribution of all the four bases at every position along the UMI sequence (Supplementary Fig. 2a-c and Supplementary Data 2). In the IMR90 libraries, which should more closely mirror the human reference genome, the fraction of aligned reads not overlapping with any of the corresponding restriction sites in the reference genome was 0.80% and 0.96% for NlaIII and HindIII, respectively, indicating that these enzymes are extremely specific. These results show that CUTseq is a valid method for preparing high-quality DNA libraries for sequencing on Illumina platforms.
CUTseq reproducibility and sensitivity
To evaluate the reproducibility of CUTseq, we first compared the DNA copy number profiles obtained with NlaIII and HindIII, at increasing resolutions ranging from 1 Mb up to 30 kb, for each of the cancer cell lines described above (Methods). The segmented copy number profiles were highly correlated between matched HindIII and NlaIII samples, at all the resolutions examined (Fig. 1b, c and Supplementary Fig. 3a, b). Each cell line showed a unique pattern of copy number alterations (CNAs), which were not correlated to the profiles of the other cell lines (Fig. 1c and Supplementary Fig. 3a, b), highlighting the specificity of CUTseq. Quantitative analysis of the profiles revealed that, at comparable sequencing depth, the read count profiles fluctuated more in the case of HindIII-digested samples, which is expected based on the lower cutting frequency of this enzyme compared with NlaIII (Supplementary Figs. 1 and 3c, and Methods). In the case of IMR90, the DNA copy number profile was flat (Supplementary Fig. 3d), as expected for normal diploid cells. To confirm the specificity of CUTseq, we assessed the amplification status of the clinically relevant ERBB2/HER2 oncogene on chromosome (chr) 17, which is amplified in BT474 and SKBR3 cells, but not in MCF7 cells, as previously shown10,11. Indeed, in BT474 and SKBR3 cells, but not in MCF7 cells, CUTseq detected a clear amplification of the ERBB2 locus, both using HindIII and NlaIII (Fig. 1d). Thus, CUTseq is able to reproducibly detect cell type-specific copy number profiles using DNA extracted from cell lines.
We then assessed the reproducibility of CUTseq in FFPE samples. To this end, we first prepared two replicate libraries for each of five FFPE tumor samples, including two colon adenocarcinomas (COAD) and three melanomas (MELA) (Supplementary Table 2, Supplementary Data 2, and Methods). DNA copy number profiles were highly similar between replicates, across multiple resolutions (Fig. 1e, f and Supplementary Fig. 4). In line with this finding, the fraction of the genome that was detected as either amplified or deleted was highly correlated between corresponding replicates (Fig. 1g). By increasing the resolution, the distribution of the length of amplified and deleted genomic segments progressively shifted towards shorter lengths in a reproducible manner (Fig. 1h, i). Zooming-in on individual chromosomes revealed that the overall copy number profile was reproducible even at 10 kb resolution, whereas new features emerged reproducibly in both replicates at higher resolution (Fig. 1j), including focal amplifications and deletions, as well as more resolved complex patterns of alterations that could not be appreciated at lower resolutions (Supplementary Fig. 5a). High correlations between copy number profiles at multiple resolutions were also seen in CUTseq libraries prepared using increasing numbers of PCR cycles (Supplementary Fig. 5b, c and Methods), suggesting that extra amplification rounds do not significantly bias the copy number profiles. Furthermore, the correlation between replicates persisted by downsampling the number of reads (Supplementary Fig. 5d and Methods), demonstrating the ability of CUTseq to reproducibly detect CNAs even at relatively low sequencing depths.
Next, we investigated the sensitivity of CUTseq for picogram inputs of gDNA (125–500 pg), which most of commercially available kits cannot achieve (Supplementary Table 3). To this end, we prepared multiplexed libraries from gDNA extracted from one breast cancer (BRCA) FFPE sample, by pooling into the same IVT reaction decreasing amounts of gDNA (1, 0.5, 0.25, and 0.125 ng) (Supplementary Table 2 and Methods). To further exclude the possibility of PCR biases, we prepared libraries using either 12, 14, or 16 PCR cycles. We then sequenced all the libraries and assessed DNA copy number profiles at various resolutions (Supplementary Data 2 and Methods). The segmented DNA copy number profiles remained extremely stable even for the 0.125 ng input and were highly correlated between each other, independently of the resolution and number of PCR cycles (Fig. 1k, l, Supplementary Fig. 6, and Supplementary Fig. 7a, b). Consistent with these observations, the overall fraction of the genome either amplified or deleted was relatively constant, independently of the gDNA input, number of PCR cycles, and resolution (Supplementary Fig. 7c), despite the fact that, as already observed in cell lines, the read count fluctuations progressively increased at higher resolutions and lower genome coverage (Supplementary Fig. 7d-f). Altogether, these results demonstrate that CUTseq is a reproducible and sensitive method that allows robust DNA copy number profiling across a broad range of resolutions, even for picogram amounts of gDNA extracted from FFPE samples.
CUTseq benchmarking
Next, we benchmarked CUTseq against standard methods of NGS library preparation. To do so, we used gDNA extracted from 10 FFPE samples representing four different tumor types, including four breast adenocarcinomas (BRCA), four COAD, two gastrointestinal stromal tumors (GIST), and two MELA samples (Supplementary Table 2 and Methods). For each sample, we constructed two libraries, one using CUTseq and the other using the commercially available library preparation kit, NEBNext® Ultra™ II (Methods). DNA copy number profiling at various resolutions (1 Mb up to 30 kb) revealed that the CUTseq and NEBNext profiles were strongly correlated, independently of the resolution (Fig. 2a, Supplementary Figs. 8 and 9, and Supplementary Fig. 10a). Consistent with this, the fraction of the genome that was detected as either amplified or deleted was highly correlated between matched samples (Fig. 2b and Supplementary Fig. 10b). Altogether, these results validate CUTseq as a sensitive and reliable method that can be used for DNA copy number profiling in FFPE samples, including low-input DNA specimens.
Compatibility of CUTseq libraries with exome capture
We then performed a proof-of-principle experiment to test whether CUTseq libraries are compatible with exome capture. To this end, we first prepared two replicate CUTseq libraries using gDNA extracted from SKBR3 cells and captured the exome using the SureSelect exome capture kit from Agilent Technologies. As a control, we prepared two replicate libraries from the same gDNA, but instead we used a commercial kit also from Agilent and captured them with the SureSelect kit (Supplementary Table 2, Supplementary Data 2, and Methods). SNV calling revealed that high-confidence SNVs (at least 50× coverage) were more concentrated around NlaIII recognition sites in CUTseq compared with Agilent samples (distance to closest NlaIII site: 77.08 ± 63.68 bp for CUTseq; 123.65 ± 142.65 bp for Agilent, mean ± SD) (Supplementary Fig. 10c), as indeed expected based on the fact that in CUTseq NlaIII was used to fragment the genome. The genomic distribution and type of high-confidence SNVs were very similar between replicates and among CUTseq and Agilent samples (Fig. 2c, d). The high-confidence SNVs (72.3%) identified by CUTseq were detected in both replicates, whereas 37.8% of all the SNVs were shared between CUTseq and Agilent (Fig. 2e, f and Methods), even though the mean coverage per SNV was lower in CUTseq (Supplementary Fig. 10d), consistent with the fact that it is a reduced representation sequencing method. Similar results were obtained using gDNA extracted from two different FFPE tumor samples (Supplementary Fig. 10e, Supplementary Table 2, and Supplementary Data 2). Altogether, these results demonstrate that CUTseq libraries are compatible with standard exome capture and can thus be used for reduced representation exome sequencing.
Multi-region tumor sequencing in FFPE tissue sections
Next, we took advantage of the high sensitivity of CUTseq to assess intratumor heterogeneity of CNAs across multiple regions of individual breast cancer tissue sections. For this purpose, we retrieved 35 archival FFPE samples from 14 patients (age of specimens: 9–27 years), including primary tumors and one or more matched metastases previously profiled by whole exome sequencing12 (Supplementary Table 2). For each tumor, we stained a 4 μm-thick section with hematoxylin–eosin and then extracted gDNA from a region L, ~7 mm2 in diameter, which was confirmed by a pathologist to contain tumor cells (Fig. 3a and Methods). We split each region into half, to produce two technical replicates, L1 and L2 (Fig. 4a). In two cases, we also captured gDNA from multiple smaller regions S, ~3 mm2 in diameter (Fig. 3a and Supplementary Fig. 11a). Accurate cell counting within 80 tumor regions of similar size in a different set of breast cancer samples revealed that, typically, such regions contain between 5000 and 25,000 cells (Supplementary Fig. 11b, c and Methods). Lastly, we extracted gDNA from the remaining material in the full tissue sections F, from which L and S regions were captured (Fig. 3a).
We separately barcoded the gDNA extracted from each region and then pooled multiple gDNAs into four libraries (Supplementary Data 2). In total, we barcoded 133 regions and sequenced each library aiming to obtain at least 200 K reads per region, which is sufficient for reliable copy number calling at 100 kb resolution. Indeed, the DNA copy number profiles of the matched L1 and L2 replicates appeared very similar (Fig. 3b), and the fraction of the genome that was detected as either amplified or deleted was highly correlated across replicates (Fig. 3c). Consistent with this observation, hierarchical clustering revealed that the profiles of matched L1 and L2 replicates always clustered together (Fig. 3d, e and Supplementary Fig. 12a), further highlighting the reproducibility of CUTseq. Typically, L regions also clustered together with the corresponding F regions (Supplementary Fig. 12a), suggesting that most of the tumor cells within a single tissue section harbor the same CNAs. These observations are in line with the notion that, in breast cancer, the majority of CNAs are acquired at an early stage during tumor evolution and therefore should be detectable across multiple tumor regions13. However, we also observed some exceptions. For example, in the metastasis-b of patient KI2, the L region showed a ~900 kb amplification on chr14q24, encompassing the RAD51B gene, which was reproducibly detected in both L1 and L2 replicates, but not in the full section (Fig. 3b, arrowhead). Similarly, two S regions in the primary tumor of patient KI14 clustered apart from all the other regions and showed numerous CNAs that were not detected in the corresponding F and L regions (Fig. 3b, e). These results highlight the importance of multi-region sequencing at high spatial resolution, to capture sub-clonal CNAs, which would otherwise go undetected when extracting gDNA from larger tissue areas.
Closer examination of the copy number profiles and hierarchical clustering trees also revealed that metastatic regions from the same tumor typically clustered together, and apart from the regions of the corresponding primary lesion (Fig. 3b-e and Supplementary Fig. 12a). Moreover, among all the regions with detectable CNAs, the metastatic regions had a significantly higher burden of amplifications and deletions compared with the primary tumor regions (P-value = 0.006, Mann–Whitney test, two-tailed) (Supplementary Fig. 12b). These results are in agreement with the findings of a recent study on a larger sample cohort, according to which breast cancer distant metastases typically show a different, although phylogenetically related, mutational landscape compared with the corresponding primary tumors, as a result of ongoing genome instability and tumor evolution14.
Finally, we checked how many of the 712 cancer-associated genes in the COSMIC database15 are affected by CNAs in different tumor regions. Two hundred and forty-one of the 712 genes (33.8%) were amplified, whereas 261 genes (36.6%) were deleted in one or more tumor sites, regions, or patients in our cohort. The top-three amplified genes were MYC, NDRG1, and RAD21, whereas KMTA, PAFAH1B2, and POU2AF1 were the three most frequently deleted genes (Fig. 3f, g and Methods). Hierarchical clustering revealed at least two major groups of samples: one group harboring amplifications and deletions in a large subset of COSMIC genes; and the other group predominantly characterized by amplifications in a smaller subset of COSMIC genes, including many genes that are recurrently affected by CNAs in breast cancer16, such as MYC, ERBB2, CCND1, MDM2, and PIK3CA (Fig. 3h and Methods). Among frequently amplified genes, MYC and ERBB2 were amplified in 7 and 8 out of 14 patients, respectively (50% and 57%), whereas, among frequently deleted genes, the classical onco-suppressor TP53 gene was deleted in 4 out of 14 patients (28.6%). Five primary tumors in which CUTseq detected HER2 amplification (KI2, 4, 10, 11, 12) were also HER2-positive based on immunohistochemistry (Supplementary Table 2), further validating our method. In one case (KI7), CUTseq detected HER2 amplification only in the metastasis, but not in the corresponding primary tumor (Fig. 3b, arrowhead), in line with recent observations that some breast cancers classified as HER2-negative might actually express HER2 at distant metastatic sites17. Overall, these results demonstrate that CUTseq is a robust and sensitive method that can be used to profile, at high spatial resolution, DNA CNAs across multiple regions in clinically relevant tumor samples, thus providing valuable insights into intratumor genetic heterogeneity.
High-throughput CUTseq
Lastly, we aimed to streamline the preparation of highly multiplexed CUTseq libraries. To reduce the assay cost and turnaround time, we developed a workflow that takes only ~8 h from DNA digestion to ready-to-sequence libraries (Fig. 4a, and Methods). To reduce reagent volumes, and therefore costs, we used a contactless liquid-dispensing robot, which allows performing digestion and ligation reactions in nanoliter volumes (Fig. 4a). As a proof-of-principle, we prepared a multiplexed library by digesting and differentially barcoding 96 replicate samples of HeLa cells gDNA inside a 96-well plate (5 ng per well) and then pooled all the samples into a single IVT reaction (Methods). We sequenced all the samples shallowly on NextSeq 500, obtaining 88 out of 96 replicates (91.7%) with at least 100 K usable reads (Fig. 4b and Methods). Notably, the sequencing error rate was very low and typically comprised between 1.5% and 1.7% (median: 1.62%; interquartile range: 1.58%–1.68%) (Fig. 4c), highlighting the precision of CUTseq, even when quick digestion and ligation are performed in nanoliter volumes. In the 88 replicates with at least 100 K usable reads, the DNA copy number profiles appeared highly similar (Fig. 3d) and were strongly correlated between each other (Fig. 4d, e and Methods). In line with this, the fraction of the genome that was detected as either amplified or deleted was very homogenous across replicates (Fig. 4f). Importantly, the cumulative cost of preparing libraries for a large number of samples is substantially lower for CUTseq compared with available commercial kits, independently of the use of a nanoliter dispensing device (Supplementary Note 1). These results demonstrate that high-throughput CUTseq is a cost-efficient method for sequencing multiple samples in parallel, including low-input gDNA samples.
Discussion
We have developed a streamlined method for gDNA barcoding and amplification, which enables the generation of multiplexed DNA sequencing libraries from both fixed and non-fixed cell and tissue samples, including single FFPE tissue sections or small regions thereof. The key advantage of CUTseq compared with standard methods of NGS library preparation is that each sample gets barcoded upfront, instead of at the end of the library preparation workflow, which allows multiple samples to be pooled together into the same library. This is possible, thanks to the combination of two widely available molecular biology tools: (i) type-II restriction enzymes that produce stereotypic DNA overhangs, to which complementary adapters can be immediately ligated without the need for end-repair, unlike what is done in most of conventional NGS library preparation methods (Supplementary Table 3); and (ii) IVT, which allows pooling together and co-amplifying multiple samples in the same reaction. Another advantage is the incorporation of UMIs9 at the site of CUTseq adapter ligation, which allows post-sequencing removal of PCR duplicates and single-molecule counting, without having to perform paired-end sequencing. Thanks to all these features, multiple samples can be merged into a single library and sequenced together without the need to prepare and quantify multiple libraries, which drastically reduces the overall cost per sample, as we demonstrate in the Supplementary Note 1. Importantly, multiplexing is not only helpful to reduce costs, but is also particularly advantageous when dealing with low-input samples for which it is challenging to prepare single-sample libraries using standard technology. As we have shown here, by pooling multiple low-input samples into the same CUTseq library, we were able to obtain very reliable DNA copy number information at kilobase resolution, even for samples of only 120 pg of FFPE gDNA, which most of the existing commercial kits for NGS library preparation cannot do (see Supplementary Table 3).
One distinguishing feature of CUTseq compared with conventional NGS library preparation methods is that it uses restriction enzymes instead of random genome fragmentation, thus providing a reduced representation of the genome. The choice of the restriction enzyme depends on the cutting frequency along the genome as well as on the desired resolution. As shown in Supplementary Figs. 3c and 7d-f, the fluctuation of read counts around the segmented genomic profiles is influenced by various parameters, including sequencing depth (genome coverage), binning size (resolution), and the cutting frequency of the restriction enzyme in use. In general, at comparable sequencing depths, profiles generated with a four-base cutter appear less noisy than profiles obtained with a six-base cutter, especially at high resolutions. However, it is critical to note that, despite increasing noise levels in the raw read count profiles, the segmented profiles are extremely stable even at high resolution and picogram DNA inputs. As a rule of thumb, we recommend using a four-base cutter such as NlaIII when high resolution is desired (<50 kb), otherwise a less expensive six-base cutter such as HindIII.
Although reduced genome representation does not prevent accurate DNA copy number calling at high resolution, as we have shown here, the same feature inherently limits the ability to detect SNVs at any position in the genome. However, as we have also demonstrated in this study, CUTseq is able to reproducibly detect a considerable fraction of high-confidence SNVs detected by a standard exome capture method and, as such, it can be used for reduced representation exome sequencing. One application of reduced representation exome sequencing would be in multi-region tumor sequencing, to detect a lower number of high-confidence SNV events, but from many more regions than currently possible, at comparable sequencing costs. This would significantly improve the ability to reconstruct a tumor’s phylogeny, by comparing CNA and SNV profiles from many regions in the same tumor. Even though in this study we have used single-end sequencing and short reads, combining a frequent cutter with paired-end sequencing and long reads should, in principle, allow for higher exome coverage. Furthermore, using a cocktail of different enzymes could also increase the exome coverage. For example, we found that over 15,000 recurrent mutations in 127 genes frequently mutated in 12 major cancer types18 are <500 bp away from the closest NlaIII recognition site (Supplementary Fig. 13a). In line with this, the mean number of NlaIII recognition sites in the exons of cancer-associated genes listed in the COSMIC database15 is 4.3 kb−1 (median = 4.2 kb−1, SD = 1.3 kb–1) (Supplementary Fig. 13b), which means that most of the cancer mutations are, at least in principle, detectable with CUTseq. Thus, CUTseq is a valuable method that expands the existing toolkit for studying cancer genomes.
Compared with other reduced representation genome-sequencing methods, such as the RAD-seq method19, which is widely used in population genetics and ecology20, CUTseq requires only one, and not two, ligation events, to barcode gDNA and amplify it by IVT. This means that, for a given gDNA fragment, the probability of getting properly ligated and barcoded is higher for CUTseq compared with RAD-seq. This is particularly advantageous, especially in cases in which the starting material is very little, as in the case of gDNA extracted from small regions within individual FFPE tissue sections. Furthermore, although in RAD-seq DNA libraries are typically prepared from individual samples20, the high-throughput CUTseq workflow described here offers a streamlined and cost-effective solution for analyzing hundreds of specimens in parallel, and thus could be very useful in ecology and population genomics applications.
Derived unique key per transaction
In cryptography, Derived Unique Key Per Transaction (DUKPT) is a key management scheme in which for every transaction, a unique key is used which is derived from a fixed key. Therefore, if a derived key is compromised, future and past transaction data are still protected since the next or prior keys cannot be determined easily. DUKPT is specified in ANSI X9.24 part 1.
Overview[edit]
DUKPT allows the processing of the encryption to be moved away from the devices that hold the shared secret. The encryption is done with a derived key, which is not re-used after the transaction. DUKPT is used to encrypt electronic commerce transactions. While it can be used to protect information between two companies or banks, it is typically used to encrypt PIN information acquired by Point-Of-Sale (POS) devices.
DUKPT is not itself an encryption standard; rather it is a key management technique. The features of the DUKPT scheme are:
- enable both originating and receiving parties to be in agreement as to the key being used for a given transaction,
- each transaction will have a distinct key from all other transactions, except by coincidence,
- if a present derived key is compromised, past and future keys (and thus the transactional data encrypted under them) remain uncompromised,
- each device generates a different key sequence,
- originators and receivers of encrypted messages do not have to perform an interactive key-agreement protocol beforehand.
History[edit]
DUKPT was invented in the late 1980s at Visa but didn’t receive much acceptance until the 1990s, when industry practices shifted towards recommending, and later requiring, that each device have a distinct encryption key.
Before DUKPT, state of the art was known as Master/Session, which required every PIN-encrypting device to be initialized with a unique master key. In handling transactions originating from devices using Master/Session key management, an unwanted side effect was the need for a table of encryption keys as numerous as the devices deployed. At a major merchant acquirer the table could become quite large indeed. DUKPT resolved this. In DUKPT each device is still initialized with a distinct key, but all of the initialization keys of an entire family of devices are derived from a single key, the base derivation key (BDK). To decrypt encrypted messages from devices in the field, the recipient need only store the BDK.
Keys[edit]
As stated above, the algorithm needs an initial single key which in the original description of the algorithm was called the super-secret key, but was later renamed to—in a more official-sounding way—Base Derivation Key (or BDK). The original name perhaps conveys better the true nature of this key, because if it is compromised then all devices and all transactions are similarly compromised.
This is mitigated by the fact that there are only two parties that know the BDK:
- the recipient of the encrypted messages (typically a merchant acquirer)
- the party which initializes the encryption devices (typically the manufacturer of the device).
The BDK is usually stored inside a tamper-resistant security module (TRSM), or hardware security module (HSM). It must remain clear that this key is not the one used to initialize the encryption device that will participate in DUKPT operations. See below for the actual encryption key generation process.
- First: A key derived from the BDK, this is known as the IPEK (Initial PIN Encryption Key)
- Second: The IPEK is then injected into the devices, so any compromise of that key compromises only the device, not the BDK. This creates yet another set of keys (inside the device) irreversibly derived from it (nominally called the Future Keys)
- Fourth: Afterwards the IPEK is then immediately discarded. NOTE: This step contradicts "Session Keys" section where it indicates that only 21 "Future Keys" are generated. The IPEK must be retained by the terminal in order generate the next batch of 21 Future Keys.
- Fifth: Future Keys are used to encrypt transactions in the DUKPT process.
Upon detection of compromise the device itself a new is derived inside the and the Derived Key Generation Process
Communication[edit]
Origination[edit]
On the originating (encrypting) end, the system works as follows:
- A transaction is initiated which involves data to be encrypted. The typical case is a customer's PIN.
- A key is retrieved from the set of “Future Keys”
- This is used to encrypt the message, creating a cryptogram.
- An identifier known as the “Key Serial Number” (KSN) is returned from the encrypting device, along with the cryptogram. The KSN is formed from the device’s unique identifier, and an internal transaction counter.
- The (cryptogram, KSN) pair is forwarded on to the intended recipient, typically the merchant acquirer, where it is decrypted and processed further.
- Internally, the device does the following:
- Increments the transaction count (using an internal counter)
- Invalidates the key just used, and
- If necessary generates more future keys
Receiving[edit]
On the receiving (decrypting) end, the system works as follows:
- The (cryptogram, KSN) pair are received.
- The appropriate BDK (if the system has more than one) is located.
- The receiving system first regenerates the IPEK, and then goes through a process similar to that used on the originating system to arrive at the same encrypting key that was used (the session key). The Key Serial Number (KSN) provides the information needed to do this.
- The cryptogram is decrypted with session key.
- Any further processing is done. For merchant acquirers, this usually means encrypting under another key to forward on to a switch (doing a “translate”), but for certain closed-loop operations may involve directly processing the data, such as verifying the PIN.
Session Keys[edit]
The method for arriving at session keys is somewhat different on the originating side as it is on the receiving side. On the originating side, there is considerable state information retained between transactions, including a transaction counter, a serial number, and an array of up to 21 “Future Keys”. On the receiving side there is no state information retained; only the BDK is persistent across processing operations. This arrangement provides convenience to the receiver (a large number of devices may be serviced while only storing one key). It also provides some additional security with respect to the originator (PIN capture devices are often deployed in security-averse environments; the security parameters in the devices are ‘distant’ from the sensitive BDK, and if the device is compromised, other devices are not implicitly compromised).
Registers Usage[edit]
Backup Registers[edit]
The following storage areas relating to key management are maintained from the time of the "Load Initial Key" command for the life of the PIN Entry Device:
Initial Key Serial Number Register (59 bits)[edit]
Holds the left-most 59 bits of the key serial number, that was initially injected into the PIN Entry Device along with the initial PIN encryption key during the "Load Initial Key" command. The contents of this register remain fixed for the service-life of the PIN Entry Device or until another "Load Initial Key" command.
Encryption Counter (21 bits)[edit]
A counter of the number of PIN encryptions that have occurred since the PIN Entry Device was first initialized. Certain counter values are skipped (as explained below), so that over 1 million PIN encryption operations are possible. Note: The concatenation (left to right) of the Initial Key Serial Number Register and the Encryption Counter form the 80-bit (20 hexadecimal digits) Key Serial Number Register.
Future Key Registers (21 registers of 34 hexadecimal digits each)[edit]
A set of 21 registers, numbered #1 to #21, used to store future PIN encryption keys. Each register includes a 2 hexadecimal digit longitudinal redundancy check (LRC) or a 2 hexadecimal digit cyclical redundancy check (CRC).
Temporary Registers[edit]
The following storage areas relating to key management are required on a temporary basis and may be used for other purposes by other PIN processing routines:
Current Key Pointer (approximately 4 hexadecimal digits)[edit]
Contains the address of that Future Key Register whose contents are being used in the current cryptographic operation. It identifies the contents of that Future Key Register whose address is contained in the Current Key Pointer.
Shift Register (21 bits)[edit]
A 21-bit register, whose bits are numbered left to right as #1 to #21. This register normally contains 20 "zero" bits and a single "one" bit. One use of this register is to select one of the Future Key Registers. The Future Key Register to be selected is the one numbered identically to the bit in the Shift Register containing the single "one".
Crypto Register-1 (16 hexadecimal digits)[edit]
A register used in performing cryptographic operations.
Crypto Register-2 (16 hexadecimal digits)[edit]
A second register used in performing cryptographic operations.
Key Register (32 hexadecimal digits)[edit]
A register used to hold a cryptographic key.
Practical Matters (KSN scheme)[edit]
In practical applications, one would have several BDKs on record, possibly for different customers, or to contain the scope of key compromise. When processing transactions, it is important for the receiver to know which BDK was used to initialize the originating device. To achieve this, the 80-bit KSN is structured into three parts: as Key Set ID, a TRSM ID, and the transaction counter. The algorithm specifies that the transaction counter is 21-bits, but treats the remaining 59 bits opaquely (the algorithm only specifies that unused bits be 0-padded to a nibble boundary, and then 'f' padded to the 80-bit boundary). Because of this, the entity managing the creation of the DUKPT devices (typically a merchant acquirer) is free to subdivide the 59 bits according to their preference.
The industry practice is to designate the partitioning as a series of three digits, indicating the number of hex digits used in each part: the Key Set ID, the TRSM ID, and the transaction counter. A common choice is '6-5-5', meaning that the first 6 hex digits of the KSN indicate the Key Set ID (i.e., which BDK is to be used), the next 5 are the TRSM ID (i.e. a device serial number within the range being initialized via a common BDK), and the last 5 are the transaction counter.
This notational scheme is not strictly accurate, because the transaction counter is 21 bits, which is not an even multiple of 4 (the number of bits in a hex digit). Consequently, the transaction counter actually consumes one bit of the field that is the TRSM ID (in this example that means that the TRSM ID field can accommodate 2(5*4-1) devices, instead of 2(5*4), or about half a million).
Also, it is common practice in the industry to use only 64-bits of the KSN (probably for reasons pertinent to legacy systems, and DES encryption), which would imply that the full KSN is padded to the left with four ‘f’ hex digits. The remaining 4 hex digits (16-bits) are available, nonetheless, to systems which can accommodate them.
The 6-5-5 scheme mentioned above would permit about 16 million BDKs, 500,000 devices per BDK, and 1 million transactions per device.
Png"divlilidivimg src"https:www. hexwar. comwp-contentuploads201606UnitRenders-190x107. png"divlilidivimg src"https:www. hexwar.
.What’s New in the Accurate ID Standard v2.5 serial key or number?
Screen Shot
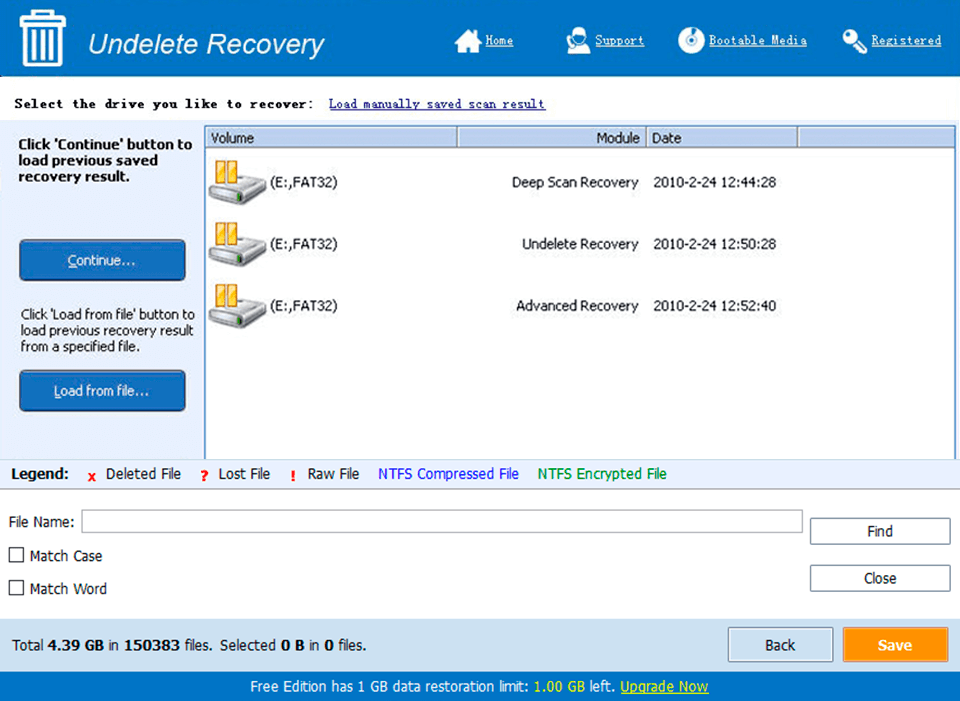
System Requirements for Accurate ID Standard v2.5 serial key or number
- First, download the Accurate ID Standard v2.5 serial key or number
-
You can download its setup from given links: